Image retrieval method based on weight learning hypergraphs and multivariate information combination
A multi-information and image retrieval technology, applied in special data processing applications, instruments, electronic digital data processing, etc., can solve problems such as the inability to adjust the structure of the hypergraph, the wrong spelling of labels, and the inability to achieve satisfactory results.
- Summary
- Abstract
- Description
- Claims
- Application Information
AI Technical Summary
Problems solved by technology
Method used
Image
Examples
Embodiment
[0104] In this embodiment, a certain network image library with user-generated tags and geographic annotation information is processed. In an embodiment of the present invention, the method includes the following steps:
[0105] Step 1: Extract multiple image features: for each network image in the image library, extract its visual spatial features, semantic spatial features, and geographic spatial features;
[0106] In this embodiment, the specific extraction process of the visual-spatial features, semantic-spatial features and geo-spatial features described in step 1 is as follows:
[0107] Step 1.1: The visual-spatial feature extraction method is as follows:
[0108] Use Gist features to describe the visual characteristics of the image, filter the image with a Gabor filter bank of 4 scales and 8 directions, and extract information in different frequencies and directions of the image;
[0109] Divide the filtered image group into 4×4 regular grids, take the mean value of th...
PUM
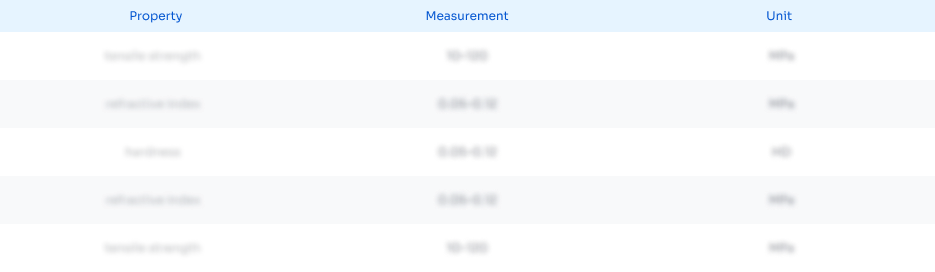
Abstract
Description
Claims
Application Information
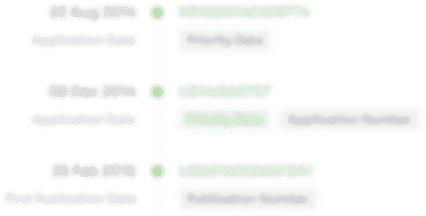
- R&D Engineer
- R&D Manager
- IP Professional
- Industry Leading Data Capabilities
- Powerful AI technology
- Patent DNA Extraction
Browse by: Latest US Patents, China's latest patents, Technical Efficacy Thesaurus, Application Domain, Technology Topic, Popular Technical Reports.
© 2024 PatSnap. All rights reserved.Legal|Privacy policy|Modern Slavery Act Transparency Statement|Sitemap|About US| Contact US: help@patsnap.com