Digital image multi-semantic annotation method based on spatial dependency measurement
A digital image, dependent technology, applied in the field of electronic information, which can solve the problem of rare semi-supervised learning methods
- Summary
- Abstract
- Description
- Claims
- Application Information
AI Technical Summary
Problems solved by technology
Method used
Image
Examples
Embodiment Construction
[0056] according to figure 1 The specific steps involved in deploying the embodiment of the present invention are as follows:
[0057] Step 1. Input 200 digital images with known semantics and the other 1800 digital images that need to be semantically annotated to the computer, including 5 types of deserts, mountains, seas, sunsets and trees; unified all image formats into RGB format, and All images are normalized to 512×512 in size; all images here are from the image database published by the Institute of Machine Learning and Data Mining, Nanjing University, available from the website http: / / lamda.nju.edu.cn / data_MIMLimage.ashx Download in;
[0058] Step 2. Use Gist descriptors to extract the global texture features of the image: convert each image into a gray image, perform Gabor filtering in 4 scales and 8 directions, and perform 4×4 partitioning on the filtered image to obtain each image. The 512-dimensional Gist feature column vector of a graph; these feature vectors form a v...
PUM
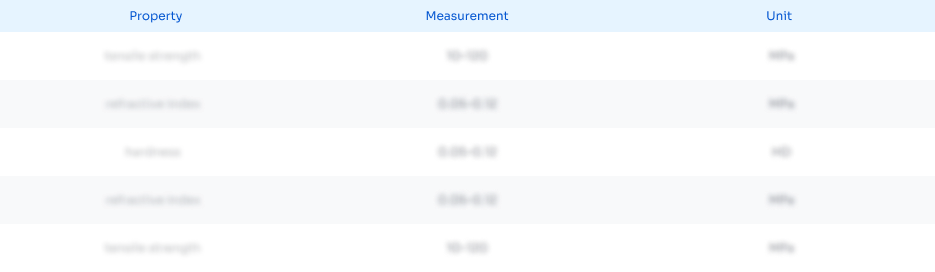
Abstract
Description
Claims
Application Information
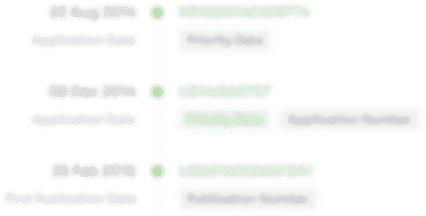
- R&D Engineer
- R&D Manager
- IP Professional
- Industry Leading Data Capabilities
- Powerful AI technology
- Patent DNA Extraction
Browse by: Latest US Patents, China's latest patents, Technical Efficacy Thesaurus, Application Domain, Technology Topic, Popular Technical Reports.
© 2024 PatSnap. All rights reserved.Legal|Privacy policy|Modern Slavery Act Transparency Statement|Sitemap|About US| Contact US: help@patsnap.com