Image data dimension reduction method based on two-dimensional kernel entropy component analysis
A technology of nuclear entropy component analysis and image data, applied in the research field of dimensionality reduction theory and application technology, can solve problems such as high computational complexity and inability to effectively use spatial structure information of image data
- Summary
- Abstract
- Description
- Claims
- Application Information
AI Technical Summary
Problems solved by technology
Method used
Image
Examples
Embodiment Construction
[0058] In order to better explain the method for reducing the dimensionality of image data based on two-dimensional nuclear entropy component analysis, the forehead images with two different expressions in the FERET face database are used for analysis, dimensionality reduction and classification.
[0059] The present invention is a method for reducing the dimensionality of image data based on two-dimensional nuclear entropy component analysis. figure 1 As shown, the specific implementation steps are as follows:
[0060] (1). Read in image data: read in FERET face database image data, the original image data size is 80 80. In this embodiment, the image data is cropped to a size of 60 60 image data;
[0061] (2). Use Parzen window to estimate the kernel function, denoted as , Where, the quadratic Renyi entropy expression:
[0062] (1)
[0063] Where Is 200 60 60 image data matrix; Is the image data matrix The probability density function of, anal...
PUM
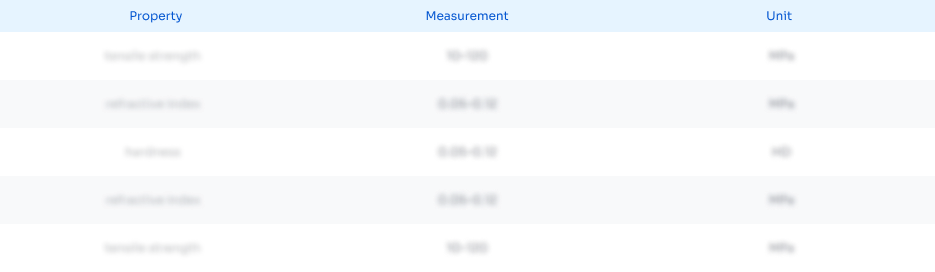
Abstract
Description
Claims
Application Information
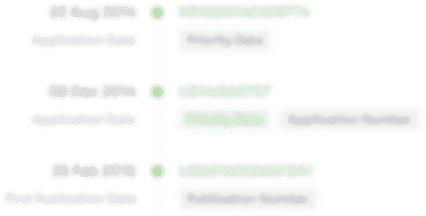
- Generate Ideas
- Intellectual Property
- Life Sciences
- Materials
- Tech Scout
- Unparalleled Data Quality
- Higher Quality Content
- 60% Fewer Hallucinations
Browse by: Latest US Patents, China's latest patents, Technical Efficacy Thesaurus, Application Domain, Technology Topic, Popular Technical Reports.
© 2025 PatSnap. All rights reserved.Legal|Privacy policy|Modern Slavery Act Transparency Statement|Sitemap|About US| Contact US: help@patsnap.com