Random forest visualized data analysis method based on largeVis
A random forest and data analysis technology, applied in the fields of big data analysis, pattern recognition, and machine learning, can solve problems such as low precision, low precision, and reduced efficiency of t-SNE, and achieve improved operating speed, good adaptability, and reliability highly usable effect
- Summary
- Abstract
- Description
- Claims
- Application Information
AI Technical Summary
Problems solved by technology
Method used
Image
Examples
Embodiment Construction
[0037] The technical solution of the present invention will be specifically described below in conjunction with the accompanying drawings.
[0038] A kind of random forest visualization data analysis method based on LargeVis of the present invention is realized according to the following steps:
[0039] Step S1: training data set preprocessing;
[0040] Step S2: extracting sample features whose proportion in the training data set is greater than the preset proportion threshold through random forest;
[0041] Step S3: Using LargeVis for dimensionality reduction
[0042] Step S4: Perform visualization processing based on Random Forest of LargeVis.
[0043] In this embodiment, due to the problems of unbalanced data samples and outliers in practical applications, this will lead to poor classification results. Imbalanced training data sets can cause many problems in pattern recognition. For example, if the dataset is unbalanced, the classifier tends to "learn" the largest propo...
PUM
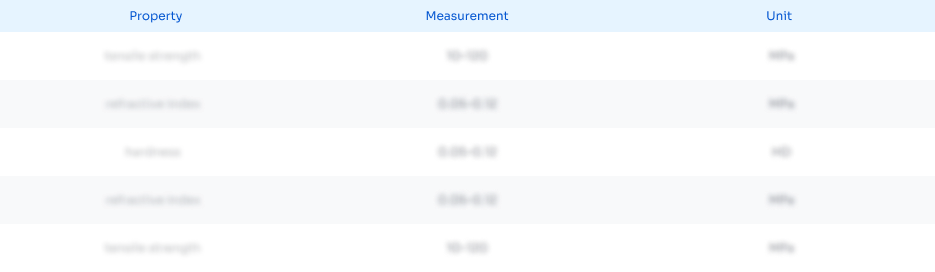
Abstract
Description
Claims
Application Information
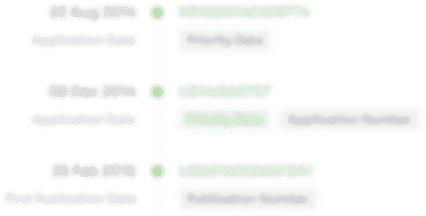
- R&D Engineer
- R&D Manager
- IP Professional
- Industry Leading Data Capabilities
- Powerful AI technology
- Patent DNA Extraction
Browse by: Latest US Patents, China's latest patents, Technical Efficacy Thesaurus, Application Domain, Technology Topic, Popular Technical Reports.
© 2024 PatSnap. All rights reserved.Legal|Privacy policy|Modern Slavery Act Transparency Statement|Sitemap|About US| Contact US: help@patsnap.com