Method for detecting P300 electroencephalogram based on convolutional neural network
A convolutional neural network and EEG signal technology, applied in the direction of user/computer interaction input/output, instrument, mechanical mode conversion, etc., can solve the problems of low signal detection accuracy, unstable system, long training time, etc. Achieve the effects of increasing classification accuracy, improving timeliness, and improving the system
- Summary
- Abstract
- Description
- Claims
- Application Information
AI Technical Summary
Problems solved by technology
Method used
Image
Examples
Embodiment Construction
[0037] The present invention will be further described below in conjunction with drawings and embodiments.
[0038]The present invention mainly draws on the idea of weight sharing and local receptive field of the convolutional neural network method. Due to the large number of weight parameters of the traditional neural network, when the number of hidden layers is large, the amount of calculation is huge, and the influence of the backpropagation method on the neural network after passing through multiple hidden layers is minimal, and the neural network cannot achieve good results. The convolutional neural network fully solves this problem through weight sharing and local receptive field ideas. Each neuron uses the same convolution kernel when convolving different feature maps, which will greatly reduce the weight parameters; at the same time, when the convolution kernel convolves the feature map, the size of the convolution kernel is designed to extract the local part of the ...
PUM
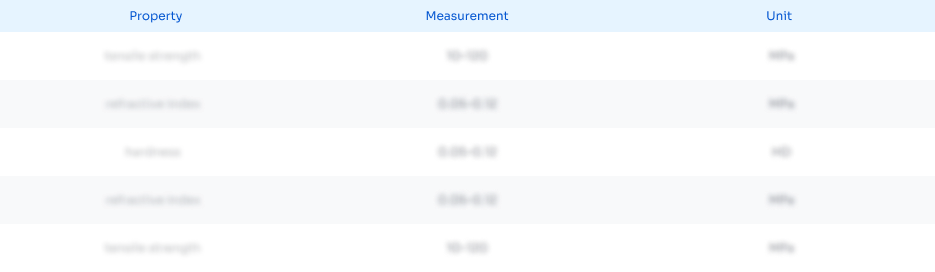
Abstract
Description
Claims
Application Information
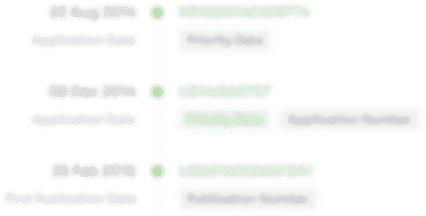
- R&D Engineer
- R&D Manager
- IP Professional
- Industry Leading Data Capabilities
- Powerful AI technology
- Patent DNA Extraction
Browse by: Latest US Patents, China's latest patents, Technical Efficacy Thesaurus, Application Domain, Technology Topic, Popular Technical Reports.
© 2024 PatSnap. All rights reserved.Legal|Privacy policy|Modern Slavery Act Transparency Statement|Sitemap|About US| Contact US: help@patsnap.com