Recommendation method in combination with frequent item set and deep learning under big data environment
A frequent itemset and deep learning technology, applied in the field of user recommendation, can solve the problems that the innovation speed of computing power cannot keep up with the growth rate of data volume, and it is difficult to handle massive data processing tasks, so as to achieve accurate user recommendation and overcome computing bottlenecks.
- Summary
- Abstract
- Description
- Claims
- Application Information
AI Technical Summary
Problems solved by technology
Method used
Image
Examples
Embodiment Construction
[0014] The present invention will be described in further detail below in conjunction with the accompanying drawings.
[0015] The recommendation method combining frequent itemsets and deep learning under the big data environment of the present invention comprises the following steps:
[0016] Step 1: Collect user behavior records, and use the MapReduce parallel computing model to mine frequent itemsets in user behavior records.
[0017] For each user User i , to record the items that the user has followed recently if , and record it in database D.
[0018] The user User i , refers to the i-th customer of the enterprise using this recommendation method, who usually pays attention to one or more products of the enterprise Item if , Item if Refers to the user User i The jth product concerned, where Item ij ∈ User i , these products express user preferences. The structure of the database D is a two-dimensional table, each row is a two-tuple, and the content is (User i ,...
PUM
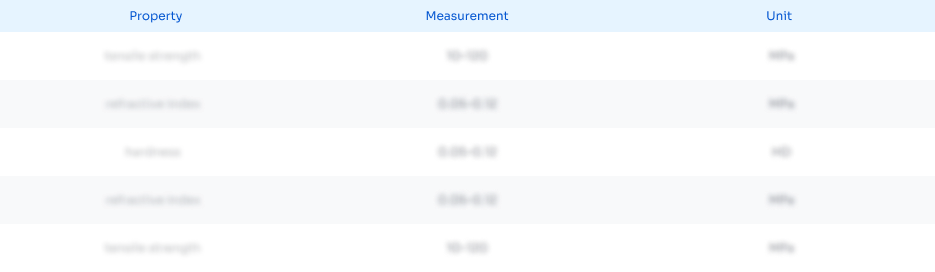
Abstract
Description
Claims
Application Information
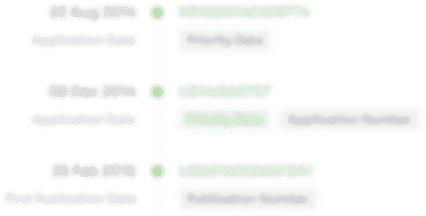
- R&D
- Intellectual Property
- Life Sciences
- Materials
- Tech Scout
- Unparalleled Data Quality
- Higher Quality Content
- 60% Fewer Hallucinations
Browse by: Latest US Patents, China's latest patents, Technical Efficacy Thesaurus, Application Domain, Technology Topic, Popular Technical Reports.
© 2025 PatSnap. All rights reserved.Legal|Privacy policy|Modern Slavery Act Transparency Statement|Sitemap|About US| Contact US: help@patsnap.com