Sample classification method based on weighted PTSVM (projection twin support vector machine)
A technology of support vector machine and classification method, which is applied in the field of non-parallel hyperplane classifiers, and can solve problems such as not considering local identification information
- Summary
- Abstract
- Description
- Claims
- Application Information
AI Technical Summary
Problems solved by technology
Method used
Image
Examples
Embodiment
[0062] like figure 1 As shown, the method includes the following steps:
[0063] Step 1: Construct a neighbor graph G within each type of sample and between different types of samples s and G d ;
[0064] The second step: according to the neighbor graph G of each type of sample s Calculate sample weights;
[0065] The third step: Calculate the weighted mean center of each type of sample on the basis of the second step;
[0066] Step 4: Determine the opposite class sample that is closer to the specific class sample according to the inter-class neighbor graph;
[0067] Step 5: Use the results of steps 1, 2, 3, and 4 to construct an optimization problem in linear mode;
[0068] Step 6: Solve the dual problem of the optimization problem in the fifth step, and obtain the decision hyperplane of the two types of samples: x T w 1 +b 1 = 0 and x T w 2 +b 2 = 0;
[0069] Step 7: Classify unknown samples according to the decision hyperplane in step 6.
[0070] For each type...
PUM
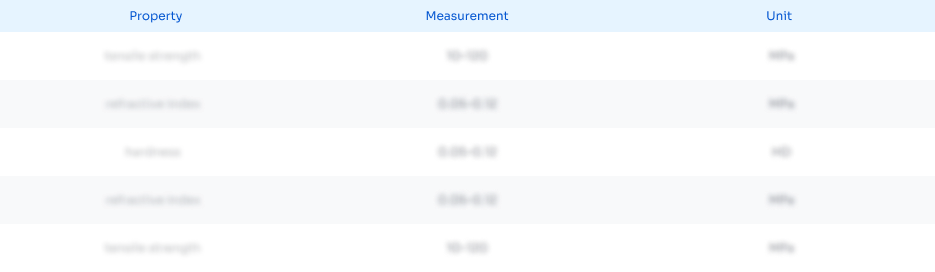
Abstract
Description
Claims
Application Information
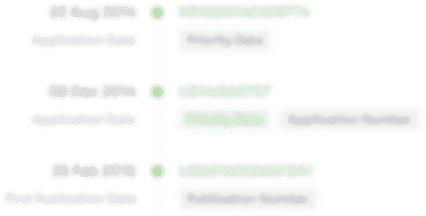
- R&D Engineer
- R&D Manager
- IP Professional
- Industry Leading Data Capabilities
- Powerful AI technology
- Patent DNA Extraction
Browse by: Latest US Patents, China's latest patents, Technical Efficacy Thesaurus, Application Domain, Technology Topic, Popular Technical Reports.
© 2024 PatSnap. All rights reserved.Legal|Privacy policy|Modern Slavery Act Transparency Statement|Sitemap|About US| Contact US: help@patsnap.com