A face recognition method based on multi-feature description and local decision weighting
A local decision-making and face recognition technology, applied in the field of pattern recognition, can solve problems such as performance degradation, ignoring the overall relationship, and not considering the vertical direction
- Summary
- Abstract
- Description
- Claims
- Application Information
AI Technical Summary
Problems solved by technology
Method used
Image
Examples
Embodiment Construction
[0092] In this example, if figure 1 As shown, a face recognition method with multi-feature description and local decision weighting includes the following steps: 1. First, use the independent component analysis algorithm to construct a global complementary subspace, and roughly classify the samples to be tested; 2. Use the proposed unified The local mean mode combines the other two texture description algorithms to construct a local complementary subspace to obtain the posterior probability value of the difficult-to-recognize sample in rough classification; 3. Set the grade score according to the posterior probability value to obtain the sample to be tested on the local complementary sub-block precise decision-making results. Specifically, proceed as follows:
[0093] Step 1. Preprocessing the face images in the face database with known labels
[0094] Using Haar-like wavelet features and integral graph method to such as Figure 2a or Figure 2b The face area in a certain fa...
PUM
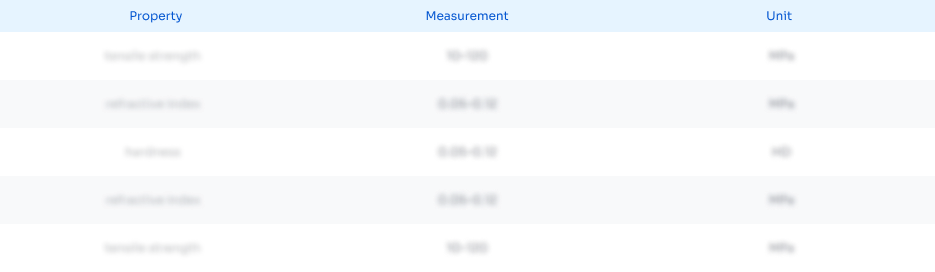
Abstract
Description
Claims
Application Information
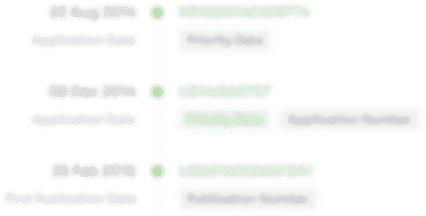
- R&D Engineer
- R&D Manager
- IP Professional
- Industry Leading Data Capabilities
- Powerful AI technology
- Patent DNA Extraction
Browse by: Latest US Patents, China's latest patents, Technical Efficacy Thesaurus, Application Domain, Technology Topic, Popular Technical Reports.
© 2024 PatSnap. All rights reserved.Legal|Privacy policy|Modern Slavery Act Transparency Statement|Sitemap|About US| Contact US: help@patsnap.com