A Sequential Detection Method of Abnormal Behavior Based on Multi-factor Inconsistency Metrics
A sequential detection, multi-factor technology, applied in instrument, calculation, character and pattern recognition, etc., can solve the problems of poor online learning effect, complex parameter settings, inaccurate statistical models, etc., and achieve high accuracy and false alarm rate. Controllable, easy-to-setup effects
- Summary
- Abstract
- Description
- Claims
- Application Information
AI Technical Summary
Problems solved by technology
Method used
Image
Examples
Embodiment Construction
[0030] Embodiments of the present invention will be described in detail below in conjunction with the accompanying drawings.
[0031] Step 1, define input and output variables:
[0032] Input variable:
[0033] 1) Abnormal threshold ε;
[0034] 2) The number k of neighbors to be considered;
[0035] 3) Training sample sequence (z 1 ,...,z l ),in
[0036] 4) Multi-factor Hausdorff distance matrix M, where each element M of the matrix i,j :i=1,...,l,j=1,...,k means z i to the sample sequence (z 1 ,...,z i-1 ,z i+1 ,...,z l ) the multi-factor Hausdorff distance between the jth nearest samples;
[0037] 5) An empty priority sequence Q;
[0038] 6) Test sample z l+1 ={x 1 ∪x 2 ∪…∪x L}, where x i ∩x j =φ:i,j=1,...,L∧j≠i;
[0039] output variable:
[0040] 1) Abnormal indicator variable in corresponding subset Calculated category, corresponding to {x 1 ∪x 2 ∪…∪x L}=z l+1 the calculated category;
[0041] 2) Distance vector (m 1 ,...,m l ), where m...
PUM
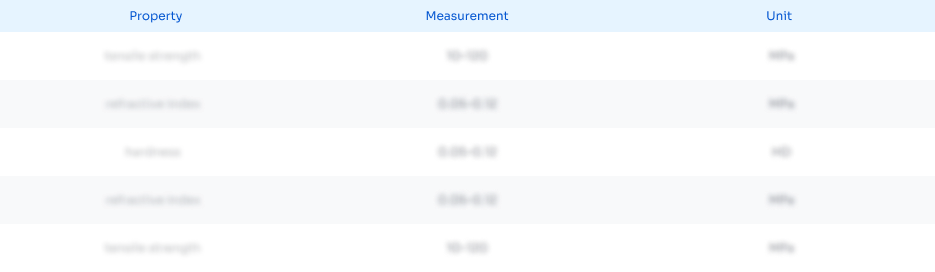
Abstract
Description
Claims
Application Information
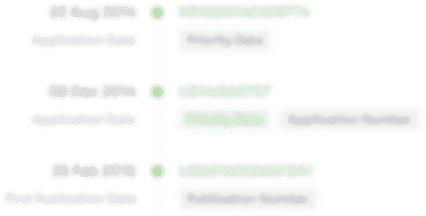
- R&D Engineer
- R&D Manager
- IP Professional
- Industry Leading Data Capabilities
- Powerful AI technology
- Patent DNA Extraction
Browse by: Latest US Patents, China's latest patents, Technical Efficacy Thesaurus, Application Domain, Technology Topic, Popular Technical Reports.
© 2024 PatSnap. All rights reserved.Legal|Privacy policy|Modern Slavery Act Transparency Statement|Sitemap|About US| Contact US: help@patsnap.com