Risk prediction method and device based on nucleus limit learning machine
A nuclear extreme learning machine and risk prediction technology, which is applied in the computer field, can solve the problems of low risk prediction accuracy and the inability to determine the optimal value of the penalty coefficient and kernel width of the nuclear extreme learning machine, so as to achieve good search ability and improve The effect of precision
- Summary
- Abstract
- Description
- Claims
- Application Information
AI Technical Summary
Problems solved by technology
Method used
Image
Examples
Embodiment 1
[0027] figure 1 It is a flow chart of a risk prediction method based on a nuclear extreme learning machine provided in Embodiment 1 of the present invention, specifically including steps S101 to S104, which are described in detail as follows:
[0028] S101. Obtain operating data of a predetermined number of enterprises, where the operating data includes a predetermined number of feature values of attribute features.
[0029] Specifically, enterprise operating data refers to the attribute characteristics of the current economic situation of the enterprise defined from the perspective of accounting. The attribute characteristics represent a series of financial ratios, which can specifically include but are not limited to cash / current liabilities (cash / current liabilities), Assets ratio (cash / total assets), current assets / current liabilities ratio (current assets / current liabilities), current assets / total assets ratio (current assets / total assets), working capital / total assets ...
Embodiment 2
[0045] figure 2 It is a flowchart of a risk prediction method based on a nuclear extreme learning machine provided in Embodiment 2 of the present invention, specifically including steps S201 to S208, which are described in detail as follows:
[0046] S201. Acquire operating data of a predetermined number of enterprises, where the operating data includes a predetermined number of feature values of attribute features.
[0047] Specifically, enterprise operating data refers to the attribute characteristics of the current economic situation of the enterprise defined from the perspective of accounting. The attribute characteristics represent a series of financial ratios, which can specifically include but are not limited to cash / current liabilities (cash / current liabilities), Assets ratio (cash / total assets), current assets / current liabilities ratio (current assets / current liabilities), current assets / total assets ratio (current assets / total assets), working capital / total assets...
Embodiment 3
[0093] image 3 It is a schematic structural diagram of an apparatus for risk prediction based on a kernel extreme learning machine provided in Embodiment 3 of the present invention. For convenience of description, only the parts related to the embodiment of the present invention are shown. image 3 An example apparatus for risk prediction based on a kernel extreme learning machine may be the subject of execution of the method for risk prediction based on a kernel extreme learning machine provided in Embodiment 1 above. image 3 The example centralized risk prediction device based on the kernel extreme learning machine mainly includes: a data acquisition module 31 , a standardization module 32 , a gray wolf optimization module 33 , a model construction module 34 and a prediction module 35 . The detailed description of each functional module is as follows:
[0094] A data acquisition module 31, configured to acquire business data of a predetermined number of enterprises, the b...
PUM
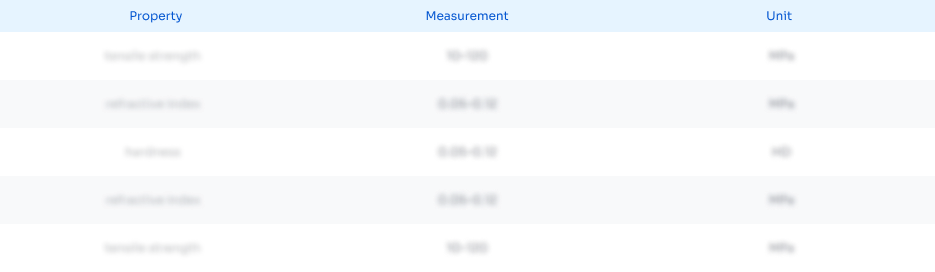
Abstract
Description
Claims
Application Information
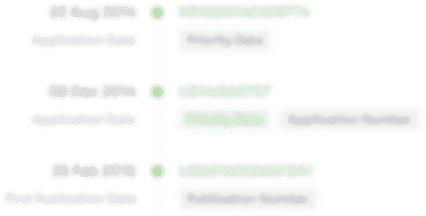
- R&D Engineer
- R&D Manager
- IP Professional
- Industry Leading Data Capabilities
- Powerful AI technology
- Patent DNA Extraction
Browse by: Latest US Patents, China's latest patents, Technical Efficacy Thesaurus, Application Domain, Technology Topic.
© 2024 PatSnap. All rights reserved.Legal|Privacy policy|Modern Slavery Act Transparency Statement|Sitemap