Wind turbine generator multivariate failure prediction method based on data driving
A technology for wind turbines and fault prediction, which is applied in data processing applications, electrical digital data processing, special data processing applications, etc., and can solve problems such as low prediction accuracy.
- Summary
- Abstract
- Description
- Claims
- Application Information
AI Technical Summary
Problems solved by technology
Method used
Image
Examples
Embodiment Construction
[0058] Based on a data-driven multivariable fault prediction method for wind turbines, this method is implemented by the following steps:
[0059] Step 1: Collect state data of the monitored wind turbine components, and extract the following feature quantities from the state data: time-domain feature quantities, frequency-domain feature quantities, and complexity feature quantities;
[0060] Step 2: Use the five-point moving average method to perform noise reduction processing on the feature quantities, thereby eliminating the random influence between the feature quantities; the noise reduction processing formula is expressed as follows:
[0061] x ′ ( 1 ) = 1 5 ...
PUM
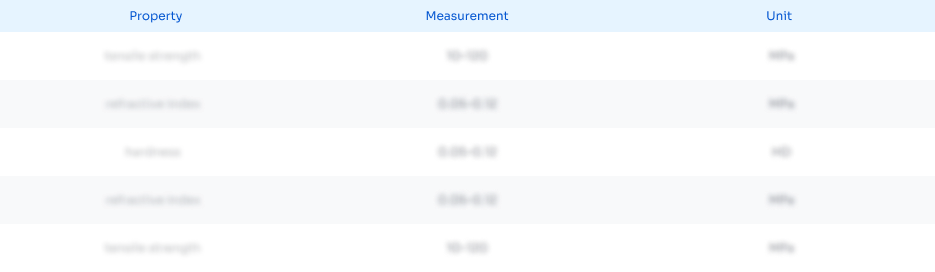
Abstract
Description
Claims
Application Information
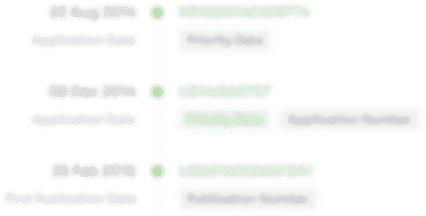
- Generate Ideas
- Intellectual Property
- Life Sciences
- Materials
- Tech Scout
- Unparalleled Data Quality
- Higher Quality Content
- 60% Fewer Hallucinations
Browse by: Latest US Patents, China's latest patents, Technical Efficacy Thesaurus, Application Domain, Technology Topic, Popular Technical Reports.
© 2025 PatSnap. All rights reserved.Legal|Privacy policy|Modern Slavery Act Transparency Statement|Sitemap|About US| Contact US: help@patsnap.com