Video human body interaction motion identification method based on optical flow graph depth learning model
A deep learning and action recognition technology, applied in the field of human interaction action recognition in video, to achieve the effect of high recognition accuracy
- Summary
- Abstract
- Description
- Claims
- Application Information
AI Technical Summary
Problems solved by technology
Method used
Image
Examples
Embodiment Construction
[0038] The preferred embodiments of the present invention are given below in conjunction with the accompanying drawings to describe the technical solution of the present invention in detail.
[0039] Such as figure 1 As shown, the present invention discloses a method for recognizing human body interaction actions in videos based on an optical flow graph deep learning model, the steps of which mainly include:
[0040] Step 1, deframe the test set video and the training set video, calculate the optical flow sequence diagram by using two adjacent frames, and obtain the optical flow sequence diagram of the test set video and the training set video;
[0041] Step 2, preprocessing the optical flow sequence diagram, deleting the optical flow diagram with less information content, retaining the optical flow diagram with more information content, and obtaining the preprocessed test set and training set optical flow sequence;
[0042] Step 3, use the training set optical flow sequence ...
PUM
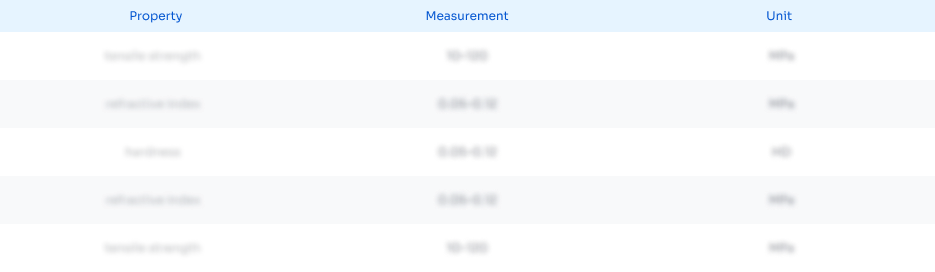
Abstract
Description
Claims
Application Information
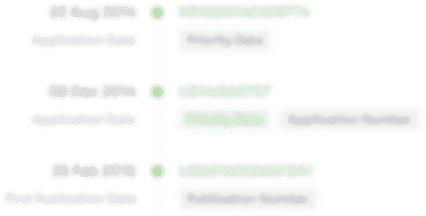
- R&D Engineer
- R&D Manager
- IP Professional
- Industry Leading Data Capabilities
- Powerful AI technology
- Patent DNA Extraction
Browse by: Latest US Patents, China's latest patents, Technical Efficacy Thesaurus, Application Domain, Technology Topic, Popular Technical Reports.
© 2024 PatSnap. All rights reserved.Legal|Privacy policy|Modern Slavery Act Transparency Statement|Sitemap|About US| Contact US: help@patsnap.com