Video image classification method based on time-space co-occurrence double-flow network
A technology of video images and classification methods, applied in biological neural network models, neural learning methods, instruments, etc.
- Summary
- Abstract
- Description
- Claims
- Application Information
AI Technical Summary
Problems solved by technology
Method used
Image
Examples
Embodiment Construction
[0042] It should be noted that, in the case of no conflict, the embodiments in the present application and the features in the embodiments can be combined with each other. The present invention will be further described in detail below in conjunction with the drawings and specific embodiments.
[0043] figure 1 It is a system flowchart of a video image classification method based on a time-space co-occurrence dual-stream network of the present invention. It mainly includes data input; spatio-temporal dual-stream network; fusion; SVM classifier.
[0044] The data input includes image and optical flow information, and the data set consists of 100 kinds of monkey video sets; the data set is divided into training set and test set. Recording monkey videos at a certain distance, this dataset has large challenges, such as large-scale camera motion changes and considerable pose changes; for each class (monkey species) the following data is provided: Video clips with active annotation...
PUM
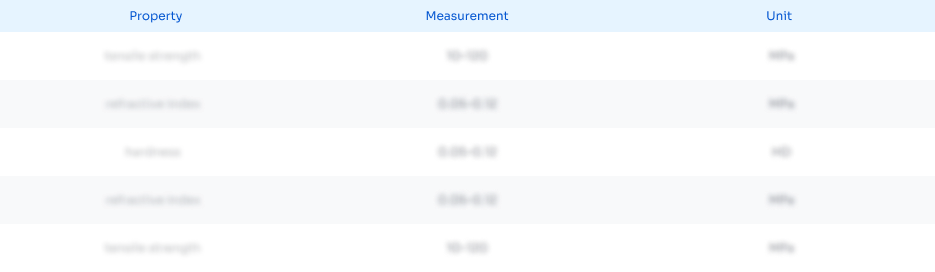
Abstract
Description
Claims
Application Information
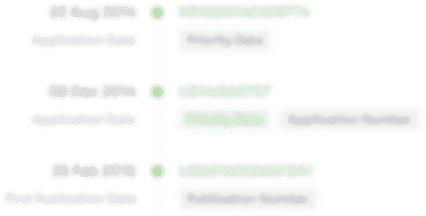
- R&D Engineer
- R&D Manager
- IP Professional
- Industry Leading Data Capabilities
- Powerful AI technology
- Patent DNA Extraction
Browse by: Latest US Patents, China's latest patents, Technical Efficacy Thesaurus, Application Domain, Technology Topic, Popular Technical Reports.
© 2024 PatSnap. All rights reserved.Legal|Privacy policy|Modern Slavery Act Transparency Statement|Sitemap|About US| Contact US: help@patsnap.com