Cost-sensitive support vector machine locomotive wheel detecting system and detecting method thereof
A support vector machine and cost-sensitive technology, applied in the field of cost-sensitive support vector machine model locomotive wheel detection system, can solve the problem of data sample misclassification cost, inequality, etc.
- Summary
- Abstract
- Description
- Claims
- Application Information
AI Technical Summary
Problems solved by technology
Method used
Image
Examples
Embodiment 1
[0085] Such as figure 1 As shown, a cost-sensitive support vector locomotive wheel state detection system includes a data preprocessing module 1, a cost-sensitive support vector machine training module 2, a parameter optimization module 3, an optimal cost-sensitive support vector machine classification module 4, and a discrimination Module 5 and wheel state output module 6;
[0086] The output terminal of the data preprocessing module 1 is connected with the input terminal of the training module 2, the output terminal of the cost-sensitive support vector machine training module 2 is connected with the input terminal of the parameter optimization module 3, and the output terminal of the parameter optimization module 3 is connected with the optimal The output end of the cost-sensitive support vector machine classification module 4 is connected, the output end of the optimal cost-sensitive support vector machine classification module 4 is connected to the input end of the discrimina...
Embodiment 2
[0097] Data imbalance refers to the large difference in the number of samples of the two categories involved in the classification, which will cause the skew of the classification hyperplane.
[0098] Figure 4 The dots in the middle circle represent the positive category, and the square dots represent the negative category. H, H1, and H2 are classification surfaces calculated based on a given sample set. Since there are very few negative class samples, some sample points that are originally negative classes are not provided, such as Figure 4 For the two square points on H4, if these two points are provided, the corresponding classification planes should be H1, H3 and H4, which is obviously very different from the previous results. Now because of the skew phenomenon, a large number of positive classes can "push" the classification toward the negative class, which affects the accuracy of the results. When the standard support vector machine is used to solve the problem of unbalan...
Embodiment 3
[0162] In order to verify the effectiveness of the method, the present invention selects a heavy-duty locomotive wheel state data set to conduct experiments to demonstrate the effectiveness and reliability of the method. In the experiment, the kernel function needs to be selected first to confirm the effectiveness of the kernel function in dealing with the binary classification problem of unbalanced data, so as to determine that the adaptive mutation particle swarm algorithm can better optimize the parameters of the cost-sensitive support vector machine classification model .
[0163] 1. Choice of kernel function
[0164] At present, in engineering practice, there are four main types of kernel functions commonly used in SVM:
[0165] 1) Linear kernel function
[0166] K(x,x i )=x T x i ;
[0167] 2) Polynomial kernel function:
[0168] K(x,x i )=(δx T x i +r) d ,δ>0;
[0169] 3) Gaussian radial basis kernel function:
[0170] K(x,x i )=exp(-||x-x i || 2 / (2δ 2 )),δ>0;
[0171] 4) Two-laye...
PUM
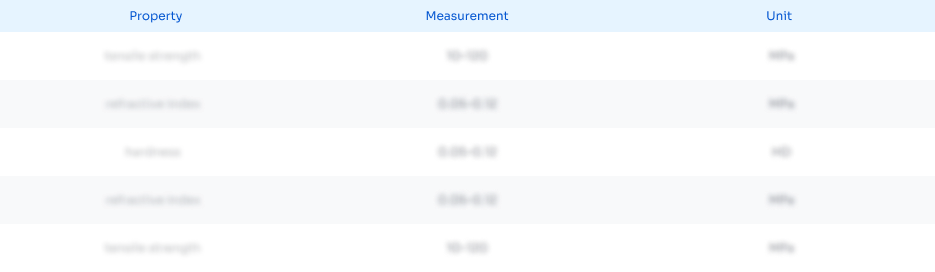
Abstract
Description
Claims
Application Information
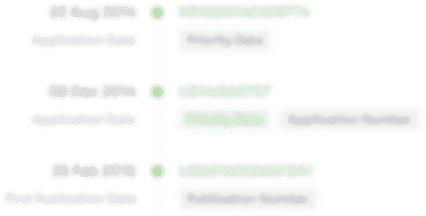
- R&D Engineer
- R&D Manager
- IP Professional
- Industry Leading Data Capabilities
- Powerful AI technology
- Patent DNA Extraction
Browse by: Latest US Patents, China's latest patents, Technical Efficacy Thesaurus, Application Domain, Technology Topic, Popular Technical Reports.
© 2024 PatSnap. All rights reserved.Legal|Privacy policy|Modern Slavery Act Transparency Statement|Sitemap|About US| Contact US: help@patsnap.com