Egg embryo classification based on deep learning
A deep learning, embryonic technology, applied in deep learning, convolutional neural network, and image processing fields, can solve problems such as low detection efficiency, false detection and missed detection, cumbersome image processing process, etc., to meet engineering requirements, high accuracy sexual effect
- Summary
- Abstract
- Description
- Claims
- Application Information
AI Technical Summary
Problems solved by technology
Method used
Image
Examples
Embodiment Construction
[0054] In order to enable your examiner to further understand the structure, features and other purposes of the present invention, it is now described in detail in conjunction with the attached preferred embodiments. The illustrated preferred embodiments are only used to illustrate the technical solutions of the present invention, not to limit the present invention. invention.
[0055] figure 1 A flow chart of the egg embryo classification method based on deep learning according to the present invention is given. The egg embryo classification method based on deep learning according to the present invention includes:
[0056] (1) Collect images of embryos on the 5th day and divide them into three types of samples according to normal embryos, aborted embryos, and infertile embryos;
[0057] (2) Preprocess the embryo image, extract the ROI area of the image and normalize the image size;
[0058] (3) Combine the transfer learning method for training, use the AlexNet classic network for...
PUM
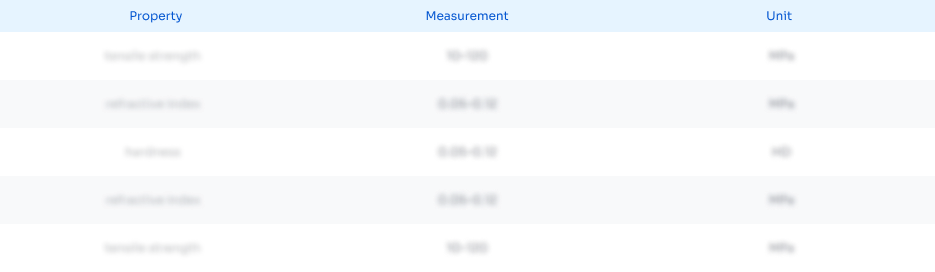
Abstract
Description
Claims
Application Information
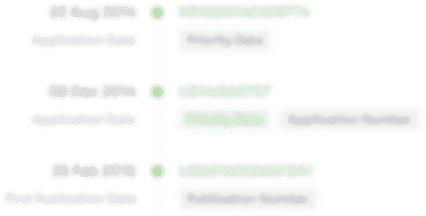
- Generate Ideas
- Intellectual Property
- Life Sciences
- Materials
- Tech Scout
- Unparalleled Data Quality
- Higher Quality Content
- 60% Fewer Hallucinations
Browse by: Latest US Patents, China's latest patents, Technical Efficacy Thesaurus, Application Domain, Technology Topic, Popular Technical Reports.
© 2025 PatSnap. All rights reserved.Legal|Privacy policy|Modern Slavery Act Transparency Statement|Sitemap|About US| Contact US: help@patsnap.com