Deep learning-based multi-view image retrieval method
A technology of image retrieval and deep learning, applied in the field of multi-view image retrieval based on deep learning, to achieve the effect of improving intuition
- Summary
- Abstract
- Description
- Claims
- Application Information
AI Technical Summary
Problems solved by technology
Method used
Image
Examples
Embodiment 1
[0031] Embodiment one, refer to figure 1 As shown, a multi-view image retrieval method based on deep learning is characterized in that the training process includes:
[0032] Step 1. Multi-view image preprocessing, normalize the multi-view image scale, normalize the image dimension, and distinguish and classify each view of the multi-view image at the same time; divide the multi-view image data set into a test data set and training data Set in two parts.
[0033] Step 2. Construct a multi-view deep convolutional neural network, use VGG-M network parameters for each type of view according to the view category, but replace the output through Softmax classification with newSoftmax, and use the pre-trained network parameters as the initial weight of the network .
[0034] Step 3. Fine-tuning the network parameters, using the training data set to reversely adjust the network parameters, and further using the labeled test data set to fine-tune the network parameters to obtain the ...
Embodiment 2
[0036] Embodiment two: reference figure 2 As shown, a multi-view image retrieval method based on deep learning is characterized in that the retrieval process includes:
[0037] Step 201. Image preprocessing, that is, to normalize the scale and dimension of the image to be retrieved.
[0038] Step 202. Pass the image through any channel of multi-view deep convolutional neural network to calculate the features of the image.
[0039] Step 203. Compare the feature of the image with the image feature in the database, output the image index number according to the distance from small to large, and extract the optimal viewing angle image corresponding to the index number from the image database.
[0040] Step 204. The search results are sorted and output by similarity, and the retrieved optimal viewing angle images and similar images are grouped and output.
Embodiment 3
[0041] Embodiment three: reference image 3 As shown, a multi-view image retrieval method based on deep learning is characterized in that,
[0042] Step 301. Normalize the image scale, and change the image to a size of 227*227.
[0043] Step 302. Image dimension normalization, if the image is RGB three-dimensional, it remains unchanged; if the image is a grayscale image or a binary image, then the increased two-dimensional image is converted into a three-dimensional image similar to RGB, and the newly increased dimension Same as the original image.
[0044] Step 303. Match each view of the image with the corresponding convolutional neural network.
[0045] In step 304. CNN, image features will be calculated through CNN convolution.
[0046] Step 305.FC2, image features are extracted through the FC2 fully connected network, and the features after FC2 extraction are 4096 dimensions.
[0047] Step 3051.Feature1, extract the feature vectors of each view after FC2 as the first ...
PUM
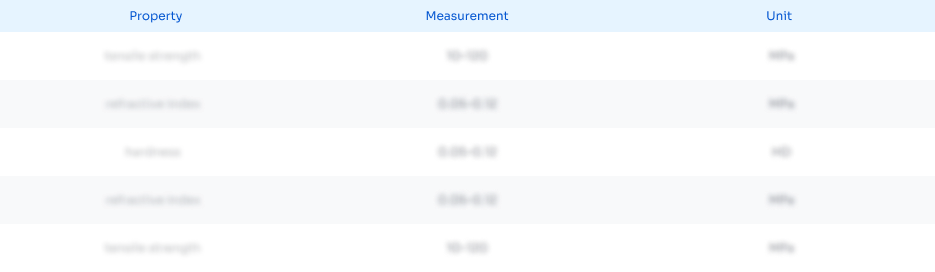
Abstract
Description
Claims
Application Information
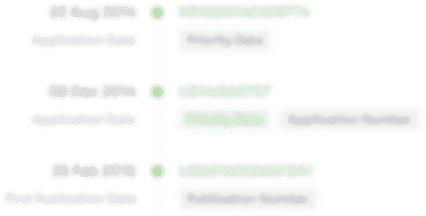
- R&D
- Intellectual Property
- Life Sciences
- Materials
- Tech Scout
- Unparalleled Data Quality
- Higher Quality Content
- 60% Fewer Hallucinations
Browse by: Latest US Patents, China's latest patents, Technical Efficacy Thesaurus, Application Domain, Technology Topic, Popular Technical Reports.
© 2025 PatSnap. All rights reserved.Legal|Privacy policy|Modern Slavery Act Transparency Statement|Sitemap|About US| Contact US: help@patsnap.com