Deep convolutional neutral network and superpixel-based image semantic segmentation method
A technology of semantic segmentation and deep convolution, applied in the field of image semantic segmentation based on deep convolutional neural network and superpixel, can solve the problem that the accuracy still needs to be improved, and achieve the effect of improving the accuracy
- Summary
- Abstract
- Description
- Claims
- Application Information
AI Technical Summary
Problems solved by technology
Method used
Image
Examples
Embodiment Construction
[0015] The image semantic segmentation method based on deep convolutional neural network and superpixels of the present invention will be further described below in conjunction with the accompanying drawings and specific embodiments: As shown in the figure, this embodiment contains the following steps:
[0016] Step 1: Train a deep convolutional network classification model from image to category label on the image classification dataset;
[0017] Step 2: Add a deconvolution layer to the deep convolutional neural network classification model, perform fine-tuning training on the image semantic segmentation dataset, and realize the mapping from the image to the image semantic segmentation result;
[0018] Step 3: Input the test image into the deep convolutional neural network semantic segmentation model to obtain the semantic label of each pixel, and at the same time send the test image to the superpixel segmentation algorithm to obtain several superpixel regions;
[0019] Step ...
PUM
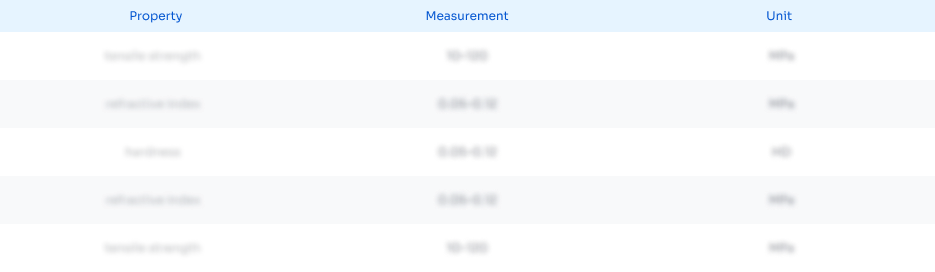
Abstract
Description
Claims
Application Information
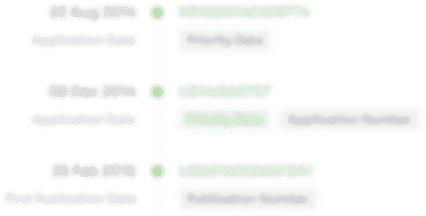
- R&D Engineer
- R&D Manager
- IP Professional
- Industry Leading Data Capabilities
- Powerful AI technology
- Patent DNA Extraction
Browse by: Latest US Patents, China's latest patents, Technical Efficacy Thesaurus, Application Domain, Technology Topic, Popular Technical Reports.
© 2024 PatSnap. All rights reserved.Legal|Privacy policy|Modern Slavery Act Transparency Statement|Sitemap|About US| Contact US: help@patsnap.com