Three-dimensional shape segmentation and semantic marking method based on projection convolutional network
A three-dimensional shape, convolutional network technology, applied in image analysis, image data processing, instrumentation, etc., can solve problems affecting the continuity and integrity of segmentation boundaries, occlusion, etc.
- Summary
- Abstract
- Description
- Claims
- Application Information
AI Technical Summary
Problems solved by technology
Method used
Image
Examples
Embodiment Construction
[0032] It should be noted that, in the case of no conflict, the embodiments in the present application and the features in the embodiments can be combined with each other. The present invention will be further described in detail below in conjunction with the drawings and specific embodiments.
[0033] figure 1 It is a system flowchart of a three-dimensional shape segmentation and semantic labeling method based on a projection convolutional network in the present invention. It mainly includes: data input; fully convolutional network (FCN) module; image surface projection layer; surface conditional random field (CRF) module; training.
[0034] The input adopts a 3D shape representation of a polygonal grid. As a preprocessing, the shape surface is sampled by uniformly distributed points (1024 are used here); the compact information points are determined to cover the shape surface to the maximum extent. For each information point, in a binary Under the perspective projection of ...
PUM
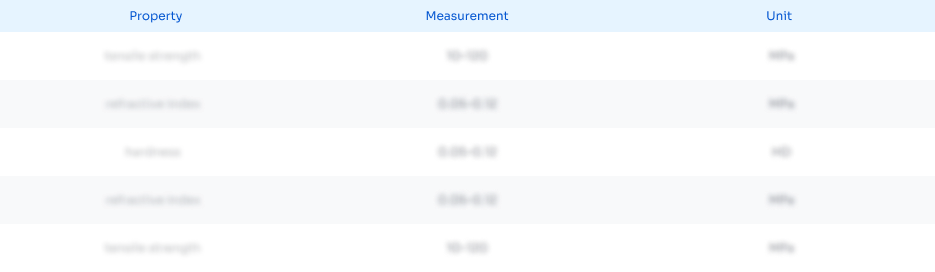
Abstract
Description
Claims
Application Information
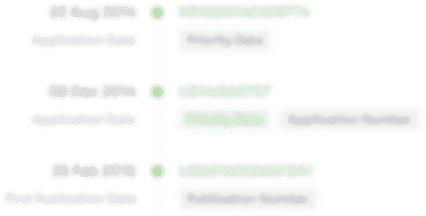
- R&D Engineer
- R&D Manager
- IP Professional
- Industry Leading Data Capabilities
- Powerful AI technology
- Patent DNA Extraction
Browse by: Latest US Patents, China's latest patents, Technical Efficacy Thesaurus, Application Domain, Technology Topic, Popular Technical Reports.
© 2024 PatSnap. All rights reserved.Legal|Privacy policy|Modern Slavery Act Transparency Statement|Sitemap|About US| Contact US: help@patsnap.com