High-resolution remote sensing image classification method based on feature pooling and divisive normalization expression
A remote sensing image and classification method technology, applied in the field of remote sensing image processing, can solve the problems of limited bag of words model, increased computational complexity, etc., and achieve the effects of reduced feature dimension, compact feature compression, and high precision
- Summary
- Abstract
- Description
- Claims
- Application Information
AI Technical Summary
Problems solved by technology
Method used
Image
Examples
Embodiment Construction
[0027] Such as figure 1 As shown, the remote sensing image is linearly filtered first, and the features of the linear filtering result are combined by feature pooling, and then the high-order correlation in the remote sensing image is reduced by denormalization, and the local descriptor is extracted in a dense grid. Finally, after Hellinger kernel mapping and feature dimensionality reduction, feature encoding is performed to form a global expression of remote sensing images, and then the classification of remote sensing images is completed after training and prediction. Specifically include the following steps:
[0028] 1) The corresponding filter response is obtained by using Log-Gabor filter and Gaussian derivative filter combined with directional amplitude.
[0029] The Log-Gabor filter includes the product of two parts to form a Log-Gabor filter. These two parts are radial filter G(ρ) and directional filter G(θ) respectively, then Where: filter center frequency ρ 0 = ...
PUM
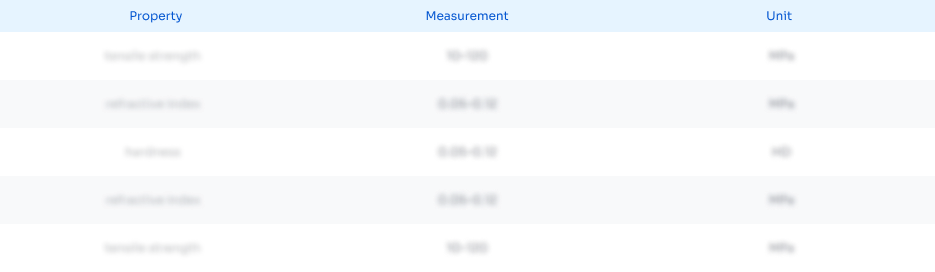
Abstract
Description
Claims
Application Information
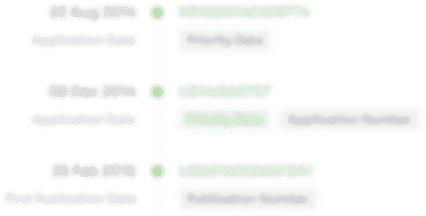
- R&D
- Intellectual Property
- Life Sciences
- Materials
- Tech Scout
- Unparalleled Data Quality
- Higher Quality Content
- 60% Fewer Hallucinations
Browse by: Latest US Patents, China's latest patents, Technical Efficacy Thesaurus, Application Domain, Technology Topic, Popular Technical Reports.
© 2025 PatSnap. All rights reserved.Legal|Privacy policy|Modern Slavery Act Transparency Statement|Sitemap|About US| Contact US: help@patsnap.com