Face recognition optimization method based on SGASEN algorithm
A face recognition and optimization method technology, applied in the direction of character and pattern recognition, calculation, gene model, etc., can solve the problems of high complexity and low recognition rate, achieve the effect of reducing complexity and improving classification accuracy
- Summary
- Abstract
- Description
- Claims
- Application Information
AI Technical Summary
Problems solved by technology
Method used
Image
Examples
Embodiment Construction
[0024] Provide the explanation of each detailed problem involved in the technical scheme of this invention below in detail:
[0025] Before proposing a new method, first of all, Zhou et al. (Zhi-Hua Zhou, Jianxin Wu, WeiTang. 263) proposed a theoretical analysis of selective ensemble learning, which mainly analyzes the selective theory for binary classification problems.
[0026] Assuming m training samples, their expected output is [d 1 , d 2 ,···,d m ] T , where d j is the expected result of the jth sample. f={f 1 ,f 2 ,···,f N} is the set of base classifiers trained by the sample set, where N is the number of base classifiers, f i is the i-th base classifier, and its classification result is [f i1 ,f i2 ,···,f im ] T , f ij That is, the output result of the i-th base classifier for the j-th sample. where d j ∈{-1,+1}(j=1,2,···,m), f ij ∈{-1,+1} (i=1,2,...,N; j=1,2,...,m). Obviously, when the i-th base classifier correctly classifies the j-th sample, there ...
PUM
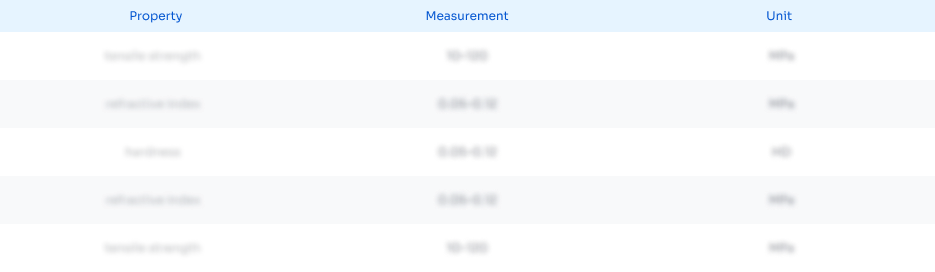
Abstract
Description
Claims
Application Information
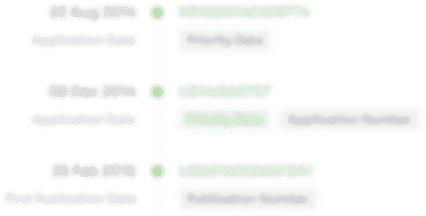
- R&D Engineer
- R&D Manager
- IP Professional
- Industry Leading Data Capabilities
- Powerful AI technology
- Patent DNA Extraction
Browse by: Latest US Patents, China's latest patents, Technical Efficacy Thesaurus, Application Domain, Technology Topic, Popular Technical Reports.
© 2024 PatSnap. All rights reserved.Legal|Privacy policy|Modern Slavery Act Transparency Statement|Sitemap|About US| Contact US: help@patsnap.com