A Manifold Regularization Correlation Filtering Target Tracking Method Based on Augmented Samples
A technology of correlation filtering and target tracking, applied in image enhancement, image analysis, instruments, etc., can solve problems such as inaccurate classification and unconsidered manifold space structure, so as to improve classification accuracy performance and discrimination ability , the effect of enriching the number of training samples
- Summary
- Abstract
- Description
- Claims
- Application Information
AI Technical Summary
Problems solved by technology
Method used
Image
Examples
Embodiment 1
[0041] S1. Extract positive base samples from the target area in the previous frame image, extract negative base samples from the non-target area, and extract unmarked base samples from the target area of the previous frame image in the current frame image; these three types Base samples form an augmented base sample set;
[0042] Wherein, the positive base samples extracted from the target area in the previous frame image, and the negative base samples extracted from the non-target area are marked base samples; the extracted base samples can be gradient histogram features, grayscale features , depth features;
[0043] During specific implementation, the image frame comes from the RGB image collected by video surveillance; one image block of the target area in the previous frame image and two image blocks of the same size adjacent to the target on the left and right sides of the target area can be extracted, and in An image block is extracted from the area where the target ...
Embodiment 2
[0097] The target tracking method is still effective if the RGB image collected by video surveillance in Embodiment 1 is changed to an infrared image collected by an infrared device, or image data obtained by other specific video collection devices or continuous image generation devices.
Embodiment 3
[0099] The present invention is still effective if the gradient histogram feature in Embodiment 1 is changed to grayscale feature, Lab color feature, HSV color feature, feature map in the neural convolutional network process, and other global image features.
PUM
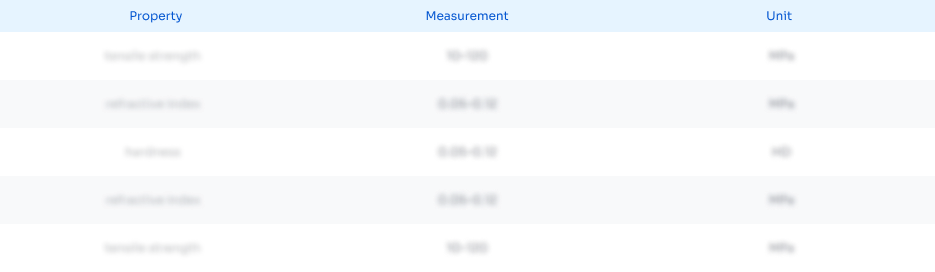
Abstract
Description
Claims
Application Information
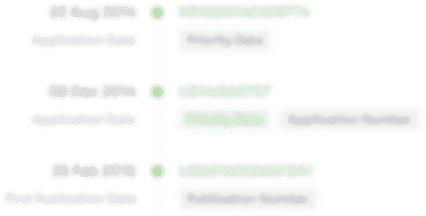
- R&D Engineer
- R&D Manager
- IP Professional
- Industry Leading Data Capabilities
- Powerful AI technology
- Patent DNA Extraction
Browse by: Latest US Patents, China's latest patents, Technical Efficacy Thesaurus, Application Domain, Technology Topic, Popular Technical Reports.
© 2024 PatSnap. All rights reserved.Legal|Privacy policy|Modern Slavery Act Transparency Statement|Sitemap|About US| Contact US: help@patsnap.com