Task splitting and mitigating learning prediction method based on multi-source domains
A prediction method and transfer learning technology, applied in the field of machine learning, to achieve the effect of preventing negative migration, avoiding under-transfer, and reducing complexity
- Summary
- Abstract
- Description
- Claims
- Application Information
AI Technical Summary
Problems solved by technology
Method used
Image
Examples
Embodiment Construction
[0034] In order to make the purpose, advantages and technical solutions of the present invention easier to be understood by those skilled in the art, so as to define and describe the present invention more clearly and in detail, the specific implementation of the present invention will be further detailed below in conjunction with experimental data and accompanying drawings elaborate.
[0035] The experimental data set used in the present invention comes from London Datastore, wherein London Datastore is a real data set disclosed by the London government. Predict the happiness of London city residents through the present invention, the target domain data set selects LondonWard Well-Being Scores, referred to as Well-Being, wherein the target domain involves 9 associated source domains, which are summarized as: Health, Economic, Safety, Education , Children, Families, Transport, Environment, Happiness. In order to make the data set adapt to the migration scenario of the present...
PUM
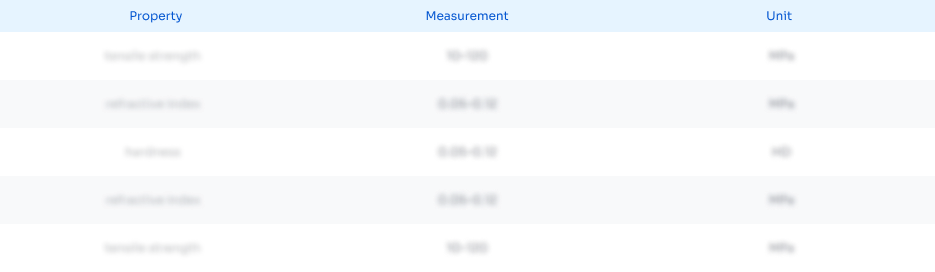
Abstract
Description
Claims
Application Information
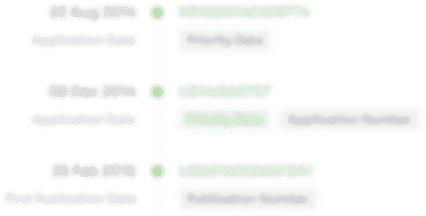
- R&D Engineer
- R&D Manager
- IP Professional
- Industry Leading Data Capabilities
- Powerful AI technology
- Patent DNA Extraction
Browse by: Latest US Patents, China's latest patents, Technical Efficacy Thesaurus, Application Domain, Technology Topic, Popular Technical Reports.
© 2024 PatSnap. All rights reserved.Legal|Privacy policy|Modern Slavery Act Transparency Statement|Sitemap|About US| Contact US: help@patsnap.com