Non-blind deblurring method for blurred image based on adaptive gradient sparse model
A blurred image and deblurring technology, applied in the field of image processing, can solve problems such as loss of intermediate frequency texture information, multiple distortions and noises, and failure to give termination iteration conditions
- Summary
- Abstract
- Description
- Claims
- Application Information
AI Technical Summary
Problems solved by technology
Method used
Image
Examples
Embodiment Construction
[0046] The present invention will be further described in detail below in conjunction with the accompanying drawings and embodiments.
[0047] A non-blind deblurring method for blurred images based on an adaptive gradient sparse model proposed by the present invention, its overall realization block diagram is as follows figure 1 shown, which includes the following steps:
[0048] ① Construct an adaptive gradient sparse regularization image deblurring model under the framework of maximum a posteriori probability:
[0049] ①_1. According to the Bayesian principle, the expression of the probability P(u|g) of obtaining a clear image u under the condition of known blurred image g is obtained: Among them, P(u|g) is also called the posterior probability of clear image u, P(g|u) represents the conditional probability of obtaining blurred image g under the condition of known clear image u, and P(g|u) is also Called the likelihood, P(u) represents the prior probability of clear image...
PUM
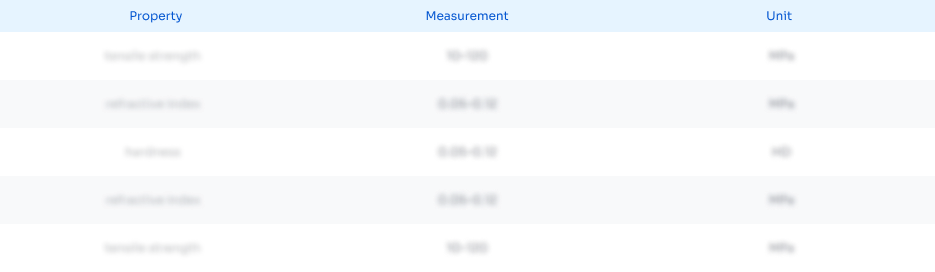
Abstract
Description
Claims
Application Information
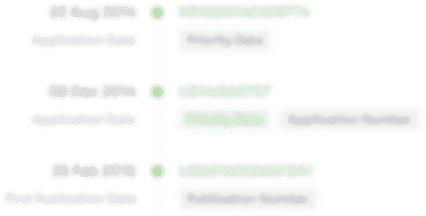
- R&D Engineer
- R&D Manager
- IP Professional
- Industry Leading Data Capabilities
- Powerful AI technology
- Patent DNA Extraction
Browse by: Latest US Patents, China's latest patents, Technical Efficacy Thesaurus, Application Domain, Technology Topic.
© 2024 PatSnap. All rights reserved.Legal|Privacy policy|Modern Slavery Act Transparency Statement|Sitemap