High-resolution SAR image classification method based on non-subsampled contourlet fully convolutional network
A non-subsampling contour, fully convolutional network technology, applied in the field of image processing, can solve the problems of high resolution SAR image difficulty, high classification accuracy, without considering the multi-scale, multi-direction and multi-resolution characteristics of high-resolution SAR images. , to avoid repeated storage and calculation of convolution, improve classification speed, and improve classification accuracy.
- Summary
- Abstract
- Description
- Claims
- Application Information
AI Technical Summary
Problems solved by technology
Method used
Image
Examples
Embodiment Construction
[0052] The present invention will be described in further detail below in conjunction with the accompanying drawings.
[0053] see figure 1 , the implementation steps of the image classification method of the present invention are as follows:
[0054] Step 1. Input the high-resolution SAR image to be classified, and perform three-layer non-subsampling contourlet transformation on each pixel to obtain its high and low frequency coefficients; the high-resolution SAR image to be classified is the German Aerospace Agency (DLR) F-SAR The X-band horizontal polarization map acquired by the aviation system in 2007, with a resolution of 1m and an image size of 6187*4278.
[0055] 1a) Transform the classification feature of each pixel point to obtain the transformation coefficient. The transformation methods include wavelet transformation, non-subsampling stationary wavelet transformation, curvelet transformation, non-subsampling contourlet transformation and other methods;
[0056] 1...
PUM
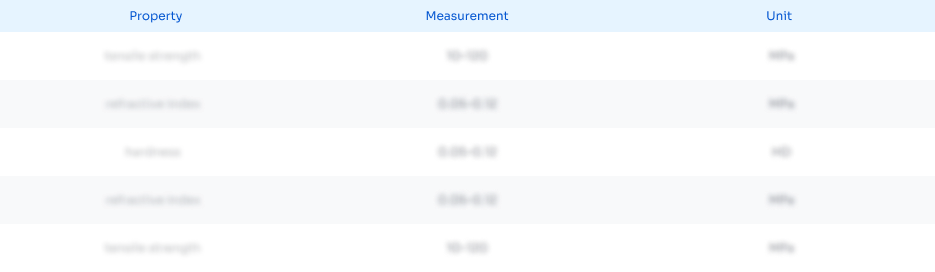
Abstract
Description
Claims
Application Information
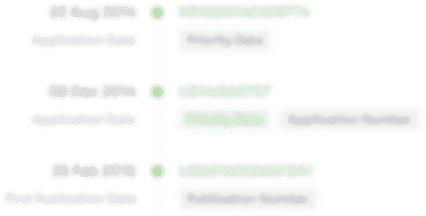
- R&D
- Intellectual Property
- Life Sciences
- Materials
- Tech Scout
- Unparalleled Data Quality
- Higher Quality Content
- 60% Fewer Hallucinations
Browse by: Latest US Patents, China's latest patents, Technical Efficacy Thesaurus, Application Domain, Technology Topic, Popular Technical Reports.
© 2025 PatSnap. All rights reserved.Legal|Privacy policy|Modern Slavery Act Transparency Statement|Sitemap|About US| Contact US: help@patsnap.com