Image super-resolution reconstruction algorithm based on shallow convolution neural network and deep convolution neural network
A convolutional neural network and super-resolution reconstruction technology, which is applied in the field of improvement combining shallow and deep networks, achieves fast reconstruction, low time complexity, and reduced ringing effects
- Summary
- Abstract
- Description
- Claims
- Application Information
AI Technical Summary
Problems solved by technology
Method used
Image
Examples
Embodiment Construction
[0033] The present invention will be described in further detail below in conjunction with the accompanying drawings.
[0034] The present invention provides a convolutional neural network combining shallow and deep networks, which extracts the image features of low-resolution sample image blocks and high-resolution sample image blocks, and learns the nonlinear mapping relationship between them. The network is trained and tested. The model widens the network, increases the number of parameters, and effectively prevents overfitting. At the same time, different two-way network structures are designed to capture different effective features, and more effective features are conducive to improving the reconstruction effect. The model in this paper is a parallel model composed of deep residual branches and shallow branches. The two inputs of the parallel network are the same LR image, and the HR image is finally obtained through the model in this paper. The basic framework of the...
PUM
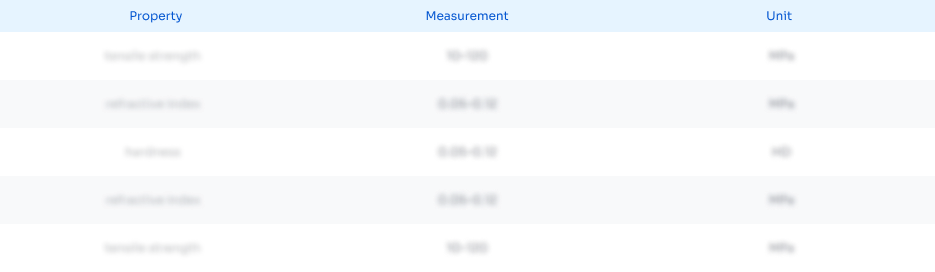
Abstract
Description
Claims
Application Information
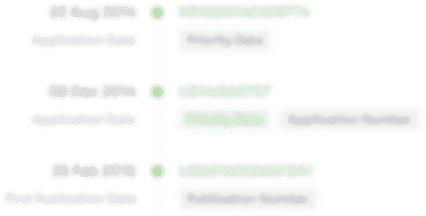
- R&D Engineer
- R&D Manager
- IP Professional
- Industry Leading Data Capabilities
- Powerful AI technology
- Patent DNA Extraction
Browse by: Latest US Patents, China's latest patents, Technical Efficacy Thesaurus, Application Domain, Technology Topic, Popular Technical Reports.
© 2024 PatSnap. All rights reserved.Legal|Privacy policy|Modern Slavery Act Transparency Statement|Sitemap|About US| Contact US: help@patsnap.com