ANFIS rule base optimization algorithm for electroencephalograph signal characteristic classification
A feature classification and EEG signal technology, applied in computing, computer components, instruments, etc., can solve problems that affect the accuracy of classification results, affect system training and learning efficiency, and improve training effects
- Summary
- Abstract
- Description
- Claims
- Application Information
AI Technical Summary
Problems solved by technology
Method used
Image
Examples
Embodiment Construction
[0022] The present invention will be described in further detail below in conjunction with the accompanying drawings: the present embodiment is implemented on the premise of the technical solution of the present invention, and detailed implementation is provided, but the protection scope of the present invention is not limited to the following embodiments.
[0023] A kind of ANFIS rule base optimization algorithm for EEG feature classification involved in the present embodiment comprises the following steps:
[0024] Step 1. Feature parameter encoding: Assume that the number of parameters used for feature parameter classification is m. Choose the Gaussian type of the input membership function, each input feature parameter contains at least one membership function, each input feature parameter membership function is at most d, and limit the upper limit of the rule base to M at the same time, set the initial feature vector as X={ x 1 ,x 2 ,...,x m}. Using the truth encoding m...
PUM
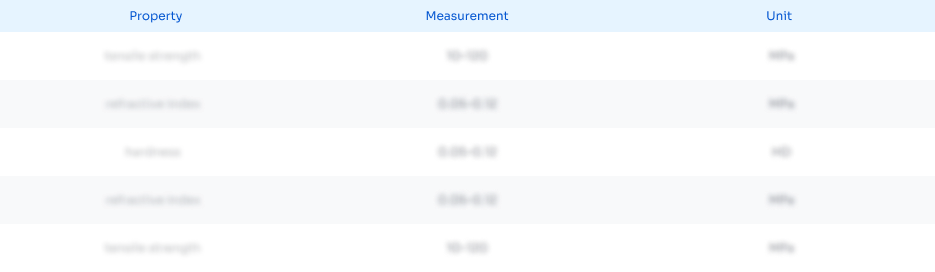
Abstract
Description
Claims
Application Information
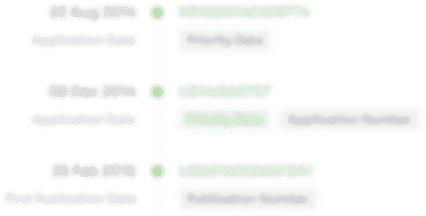
- R&D Engineer
- R&D Manager
- IP Professional
- Industry Leading Data Capabilities
- Powerful AI technology
- Patent DNA Extraction
Browse by: Latest US Patents, China's latest patents, Technical Efficacy Thesaurus, Application Domain, Technology Topic, Popular Technical Reports.
© 2024 PatSnap. All rights reserved.Legal|Privacy policy|Modern Slavery Act Transparency Statement|Sitemap|About US| Contact US: help@patsnap.com