Deep transfer learning-based unbalanced classification ensemble method
A transfer learning and classification integration technology, applied in the fields of deep learning, transfer learning and unbalanced classification, can solve the problems of consuming a lot of time and space, unable to realize unbalanced classification, unbalanced data classification, etc., to improve classification performance, Save time cost and calculation cost, improve recognition effect
- Summary
- Abstract
- Description
- Claims
- Application Information
AI Technical Summary
Problems solved by technology
Method used
Image
Examples
Embodiment Construction
[0055] The present invention will be further described below in conjunction with specific examples.
[0056] Taking the identification of the number "1" in the Chars74K data set as an example, the unbalanced deep migration integration method EUDT of the present invention is described in detail. The framework of the unbalanced deep migration integration method described in this embodiment is as follows figure 1 As shown, the specific situation is as follows:
[0057] In step 1), the sample of the number "1" in the Chars74K dataset is set as the positive class (102) of the target data, and the rest of the pictures are set as the negative class (918) of the target data. Select the data MNIST data set related to the target task in the existing public data set as the auxiliary data, set the sample of the number "1" in the MNIST data set as the positive class of the auxiliary data (6742), and set the rest of the pictures as the negative class of the auxiliary data. classes (53258)....
PUM
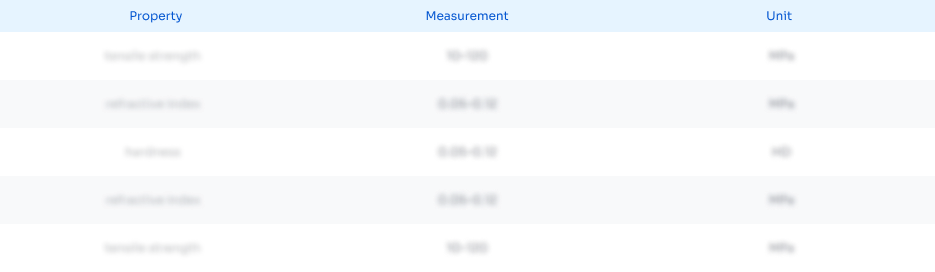
Abstract
Description
Claims
Application Information
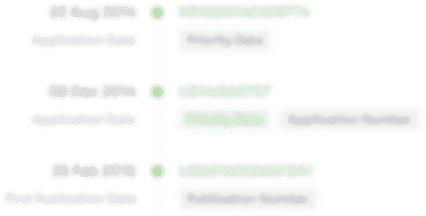
- R&D Engineer
- R&D Manager
- IP Professional
- Industry Leading Data Capabilities
- Powerful AI technology
- Patent DNA Extraction
Browse by: Latest US Patents, China's latest patents, Technical Efficacy Thesaurus, Application Domain, Technology Topic, Popular Technical Reports.
© 2024 PatSnap. All rights reserved.Legal|Privacy policy|Modern Slavery Act Transparency Statement|Sitemap|About US| Contact US: help@patsnap.com