Medical image segmentation method based on FCM algorithm in fusion with improved GSA algorithm
A medical image and algorithm technology, applied in image analysis, image enhancement, image data processing and other directions, can solve the problems of not considering the spatial structure information of the image, poor noise resistance of the algorithm, and sensitivity to noise.
- Summary
- Abstract
- Description
- Claims
- Application Information
AI Technical Summary
Problems solved by technology
Method used
Image
Examples
Embodiment Construction
[0072] Below in conjunction with accompanying drawing and specific embodiment the present invention is described in further detail:
[0073] A medical image segmentation method based on FCM algorithm fusion and improved GSA algorithm, including the following steps:
[0074] S1 uses the FCM algorithm to segment the initial medical image and initialize the clustering center
[0075] Suppose X={x 1 ,x 2 ,...x n} represents a series of data points, n is the number of pixels in the medical image, c is the number of clusters, and m is the fuzzy weighted index, which is used to control the fuzzy degree of data division;
[0076] The above data points are clustered and grouped, and the values of c and m are selected, where 2
[0077] Set the iteration stop threshold ε, ε is the convergence accuracy of medical image segmentation, where ε>0; set the number of iterations to 0, initialize the clustering center V, V={v 1 ,v 2 ...v i},v i is the i-th cluster center, i=...
PUM
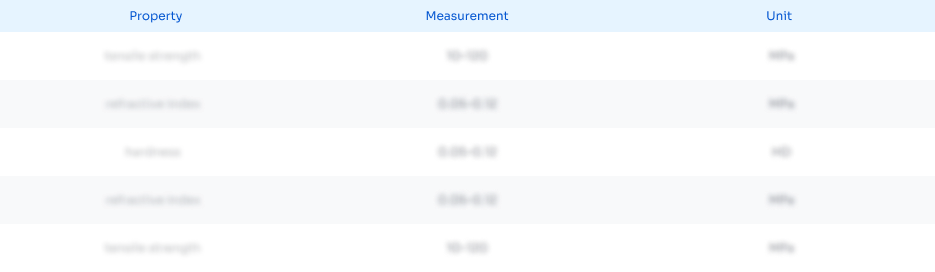
Abstract
Description
Claims
Application Information
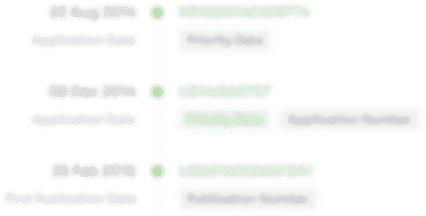
- R&D
- Intellectual Property
- Life Sciences
- Materials
- Tech Scout
- Unparalleled Data Quality
- Higher Quality Content
- 60% Fewer Hallucinations
Browse by: Latest US Patents, China's latest patents, Technical Efficacy Thesaurus, Application Domain, Technology Topic, Popular Technical Reports.
© 2025 PatSnap. All rights reserved.Legal|Privacy policy|Modern Slavery Act Transparency Statement|Sitemap|About US| Contact US: help@patsnap.com