Tensorized embedded hyperspectral image classification method based on sparse low-rank regular graph
A hyperspectral image and classification method technology, applied in the field of hyperspectral image classification based on quantitative embedding of sparse low-rank regular graphs, can solve the problems of classification result error, difficulty of multi-manual labeling samples, and neighborhood information affecting image classification effect, etc. , to achieve the effect of accurate classification
- Summary
- Abstract
- Description
- Claims
- Application Information
AI Technical Summary
Problems solved by technology
Method used
Image
Examples
Embodiment Construction
[0035] The present invention will be further described below in conjunction with the accompanying drawings.
[0036] Refer to attached figure 1 , the realization steps of the present invention are as follows.
[0037] Step 1, input hyperspectral image.
[0038] Input the hyperspectral image to be classified, and set each pixel in the input hyperspectral image as a sample.
[0039] Step 2, determine the training sample set and test sample set.
[0040] In the hyperspectral image sample set, randomly select 5% of the samples in each category as the hyperspectral image training sample set; the remaining 95% of the samples are used as the hyperspectral image test sample set.
[0041] Step 3, construct the adjacency matrix of the training sample set:
[0042] Select two samples from the training sample set, and calculate the weight between the two selected samples.
[0043] The weight between the two samples is calculated according to the following formula:
[0044]
[004...
PUM
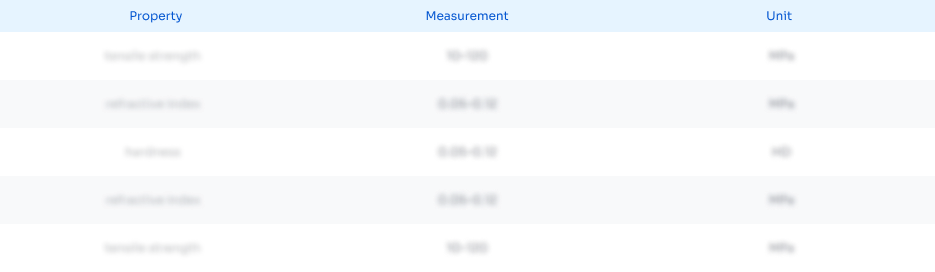
Abstract
Description
Claims
Application Information
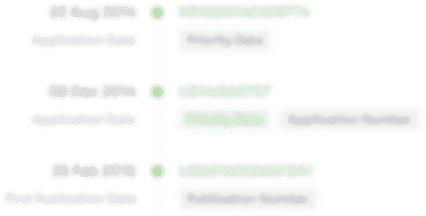
- R&D Engineer
- R&D Manager
- IP Professional
- Industry Leading Data Capabilities
- Powerful AI technology
- Patent DNA Extraction
Browse by: Latest US Patents, China's latest patents, Technical Efficacy Thesaurus, Application Domain, Technology Topic, Popular Technical Reports.
© 2024 PatSnap. All rights reserved.Legal|Privacy policy|Modern Slavery Act Transparency Statement|Sitemap|About US| Contact US: help@patsnap.com