Image semantic segmentation method adopting inverse attention and pixel similarity learning
A technique for semantic segmentation and attention, applied in the field of computer vision
- Summary
- Abstract
- Description
- Claims
- Application Information
AI Technical Summary
Problems solved by technology
Method used
Image
Examples
Embodiment 1
[0077] Embodiment 1: On the PACALConT1exT1 data set, use a kind of image semantic segmentation method that refers to inverse attention and pixel similarity learning according to the present invention and FCN-8s, BoxSup, ConT1exT1, VeryDeep, DeepLabv2-ASPP, RefineNeT1-101 Compared with RefineNeT1-152, HolisT1ic, and ModelA2, the experimental results are shown in Table 1:
[0078]
[0079]
[0080] Table 1 Comparison results of different semantic segmentation methods on the PASCALConT1exT1 test set
[0081] As can be seen from Table 1, the comparison results on the PASCALConT1exT1 test set, a kind of image semantic segmentation method that refers to inverse attention and pixel similarity learning according to the present invention has three points in terms of pixel accuracy, average pixel accuracy and average IoU accuracy. In terms of all indicators, it is better than other methods, and the accuracy has increased by about 1%.
[0082] On the PACALPerson-ParT1 data set, an...
Embodiment 2
[0086] Embodiment 2: On the NYUDv2 data set, an image semantic segmentation method using inverse attention and pixel similarity learning according to the present invention and GupT1aeT1al, FCN-32s, ConT1exT1, HolisT1ic, RefineNeT1, DeepLabv2-ASPP, DeepLab-LFOV For comparison, the experimental results are shown in Table 3:
[0087]
[0088]
[0089] Table 3 Comparison results of various semantic segmentation methods on the NYUDv2 test set
[0090] It can be seen from Table 3 that an image semantic segmentation method using inverse attention and pixel similarity learning according to the present invention obtains a better average IoU accuracy, reaching 42.1%.
Embodiment 3
[0091] Embodiment 3: On the MIT1ADE20K data set, use a kind of image semantic segmentation method that refers to inverse attention and pixel similarity learning according to the present invention and FCN-8s, DilaT1edNeT1, DilaT1edNeT1Cascade, HolisT1ic, PSPNeT1, DeepLabv2-ASPP, DeepLab -LFOV for comparison, the experimental results are shown in Table 4:
[0092]
[0093] Table 4 Comparison results of various semantic segmentation methods on the MIT1ADE20K test set
[0094] It can be seen from Table 4 that since the MIT1ADE20K data set is relatively large and the data set contains samples in various complex scenarios, it is difficult to perform semantic segmentation on the data set. From the comparison results, it can be seen that a reference inverse attention method described in the present invention Compared with the existing best method PSPNeT1, the image semantic segmentation method based on force and pixel similarity learning has improved by about 1%.
[0095] An image...
PUM
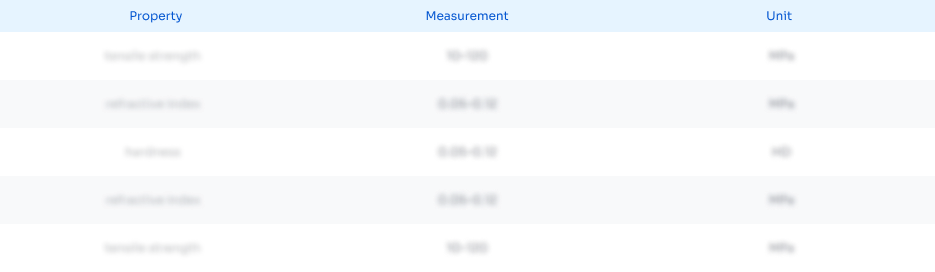
Abstract
Description
Claims
Application Information
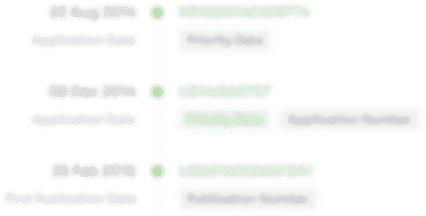
- R&D
- Intellectual Property
- Life Sciences
- Materials
- Tech Scout
- Unparalleled Data Quality
- Higher Quality Content
- 60% Fewer Hallucinations
Browse by: Latest US Patents, China's latest patents, Technical Efficacy Thesaurus, Application Domain, Technology Topic, Popular Technical Reports.
© 2025 PatSnap. All rights reserved.Legal|Privacy policy|Modern Slavery Act Transparency Statement|Sitemap|About US| Contact US: help@patsnap.com