Electric power telecommunication network device fault prediction method based on improved LSTM
A technology for power communication network and equipment failure, applied in the direction of electrical components, data exchange network, digital transmission system, etc., can solve problems such as poor prediction accuracy, achieve good sequence-related prediction problems, solve sequence-related prediction problems, and facilitate Learning the effects of long-term dependence
- Summary
- Abstract
- Description
- Claims
- Application Information
AI Technical Summary
Problems solved by technology
Method used
Image
Examples
Embodiment Construction
[0039] The technical solution proposed by the present invention can be implemented using relatively mature deep learning open source frameworks, such as TensorFlow, Torch, Caffe, Theano, etc. These frameworks have been widely used and achieved excellent results. The following drawings and examples illustrate the technical solutions of the present invention.
[0040] One, at first introduce the method principle of the present invention.
[0041] Step 1: The power communication network itself has accumulated a large amount of data, especially the log alarm data related to equipment, but these data have a lot of noise and redundant data, analysis of the characteristics of the alarm data, and the research on the distribution of these data are useful Help us filter out some illegal and noise data. In addition, the temperature and humidity data of the computer room where the equipment is located are collected, and the missing values are replaced by the nearest neighbor data. The ...
PUM
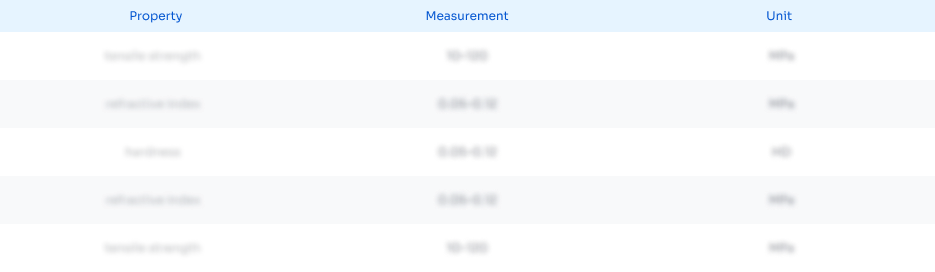
Abstract
Description
Claims
Application Information
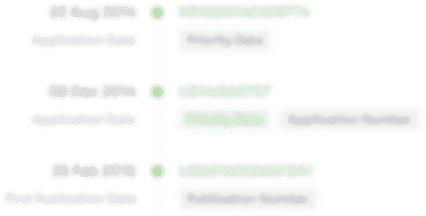
- R&D Engineer
- R&D Manager
- IP Professional
- Industry Leading Data Capabilities
- Powerful AI technology
- Patent DNA Extraction
Browse by: Latest US Patents, China's latest patents, Technical Efficacy Thesaurus, Application Domain, Technology Topic, Popular Technical Reports.
© 2024 PatSnap. All rights reserved.Legal|Privacy policy|Modern Slavery Act Transparency Statement|Sitemap|About US| Contact US: help@patsnap.com