Block sparse representation mode and structure dictionary-based hyperspectral image compression method
A technology of hyperspectral image and compression method, which is applied in the field of hyperspectral image compression based on block sparse expression mode and structure dictionary, can solve the problems of high complexity, high computational complexity, and low structural similarity of reconstructed images, and achieve Effects of increasing calculation speed, shortening encoding time, and shortening reconstruction time
- Summary
- Abstract
- Description
- Claims
- Application Information
AI Technical Summary
Problems solved by technology
Method used
Image
Examples
Embodiment Construction
[0053] The compression process of the present invention will be described in detail below in combination with examples and accompanying drawings.
[0054] The 400×400×32 image is used as the training image, and the 256×256×32 image is used as the test image. The main steps include:
[0055] 1. Training offline dictionary:
[0056] 1.1 For an image of 400×400×32 (spatial resolution is 400×400, the number of bands is 32), each band contains 400×400=160000 pixels, and each pixel is arranged in a column to form training image data
[0057] 1.2 Since the number of atoms in the redundant dictionary is usually greater than or equal to 3 / 2 times the number of bands, this embodiment selects the number of atoms K=126, and uses the block sparse dictionary learning algorithm to obtain the training dictionary and the atomic block d; the objective function of the structure dictionary is:
[0058]
[0059] ||Y 0 -DX 0 || F means Y 0 -DX 0 F-norm of , then ||Y-DX|| F ;k represen...
PUM
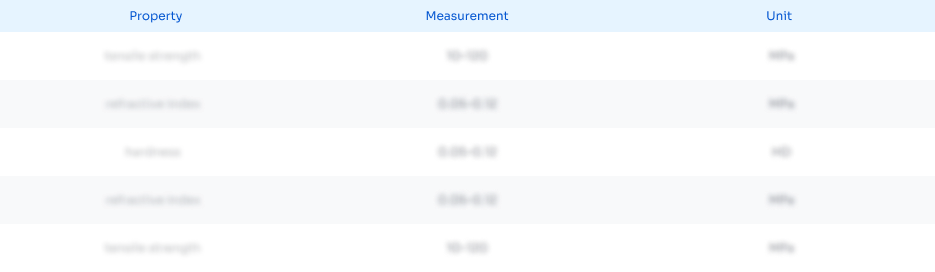
Abstract
Description
Claims
Application Information
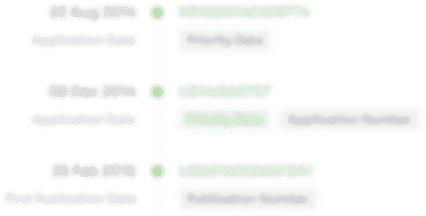
- R&D Engineer
- R&D Manager
- IP Professional
- Industry Leading Data Capabilities
- Powerful AI technology
- Patent DNA Extraction
Browse by: Latest US Patents, China's latest patents, Technical Efficacy Thesaurus, Application Domain, Technology Topic, Popular Technical Reports.
© 2024 PatSnap. All rights reserved.Legal|Privacy policy|Modern Slavery Act Transparency Statement|Sitemap|About US| Contact US: help@patsnap.com