Knowledge-graph representation learning method based on multiple semantemes
A knowledge map and learning method technology, applied in semantic analysis, structured data retrieval, instrumentation, etc., can solve problems such as inability to accurately express connections, and achieve the effect of solving heterogeneity and imbalance and good practicability
- Summary
- Abstract
- Description
- Claims
- Application Information
AI Technical Summary
Problems solved by technology
Method used
Image
Examples
Embodiment Construction
[0032] In order to more clearly illustrate the embodiments of the present invention or the technical solutions in the prior art, the following will refer to and give examples to describe the present invention in more detail.
[0033] In the prior art, only the difference between entities and relations under the same semantic relationship type is considered, and the different semantics of the relationship in the triple structure information is not fully considered, and there are many learning parameters, so it cannot be accurately represented The connection between entities and relations is also not well applied to large-scale knowledge graphs. The present invention fully considers the different semantics of the relationship in the triple structure information of the knowledge map, and defines the relationship matrix M according to the different semantics of the relationship r . And the knowledge is expressed in the form of a typical (entity 1, relation, entity 2) triple, and ...
PUM
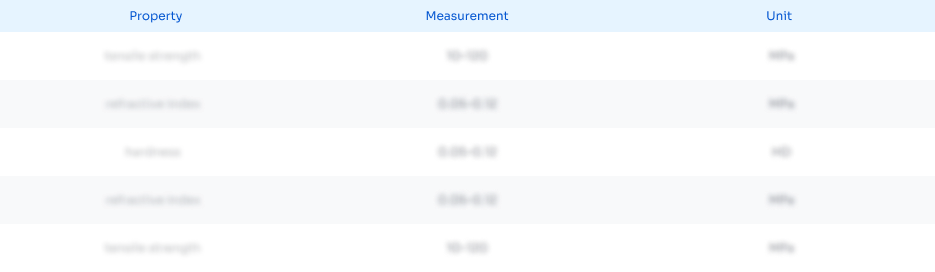
Abstract
Description
Claims
Application Information
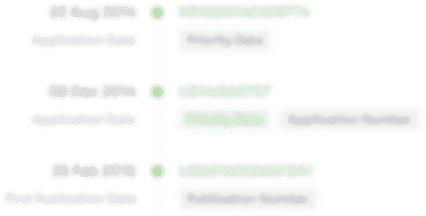
- R&D
- Intellectual Property
- Life Sciences
- Materials
- Tech Scout
- Unparalleled Data Quality
- Higher Quality Content
- 60% Fewer Hallucinations
Browse by: Latest US Patents, China's latest patents, Technical Efficacy Thesaurus, Application Domain, Technology Topic, Popular Technical Reports.
© 2025 PatSnap. All rights reserved.Legal|Privacy policy|Modern Slavery Act Transparency Statement|Sitemap|About US| Contact US: help@patsnap.com