Video abstract method based on supervised video segmentation
A technology of video summarization and video segmentation, applied in the field of video summarization of multimedia social networking, can solve the problems of not considering video structure, high similarity of summaries, and inability to display actions, so as to improve accuracy and interest, and improve efficiency and accuracy. Effect
- Summary
- Abstract
- Description
- Claims
- Application Information
AI Technical Summary
Problems solved by technology
Method used
Image
Examples
Embodiment 1
[0033] In order to solve the above problems, research methods that can comprehensively capture the structural information and similarity information of the training set videos, improve the accuracy of video segmentation and abstraction, and the degree of interest are needed.
[0034] Studies have shown that similar videos have similar structures, which can be passed to the test video by capturing the structured information of the training video, and the segmentation and summary of the test video can be known as the structured information. Embodiments of the present invention propose a video summary learning method based on supervised video segmentation, see figure 1 , see the description below:
[0035] 101: Obtain the kernel matrix of the test video through the similarity matrix and the kernel matrix of the training video, and use the kernel matrix as a regularized Laplacian matrix for time-domain subspace clustering;
[0036] 102: Introduce the time-domain Laplace regulariz...
Embodiment 2
[0047] The scheme in embodiment 1 is further introduced below in conjunction with specific calculation formulas and examples, see the following description for details:
[0048] 201: to N 1 frames of the training video and N 2 The test video of the frame, extract the color histogram feature (512 dimensions) respectively, construct a N 2 *N 1 The similarity matrix S k ;
[0049] Among them, the similarity matrix S k elements in Calculated, v i and v k are the color histogram features of the test and training videos; σ is a positive adjustable parameter; i is the index of the i-th frame of the video; k is the index of the k-th frame of the video.
[0050] 202: Get the kernel matrix L of the training video k , the kernel matrix L k Frame score matrix by user's review Obtained by diagonalization;
[0051] gt_score is the user's score for each frame of the video, for example: a video with 950 frames, gt_score is a column matrix of 950*1, which is the information of th...
Embodiment 3
[0072] Combined with the specific calculation formula, the appended figure 2 and 3 The scheme in embodiment 1 and 2 is carried out feasibility verification, see the following description for details:
[0073] The database used in this experiment is SumMe. The SumMe database consists of 25 videos with an average length of 2 minutes and 40 seconds. Each video is edited and summarized by 15 to 18 people, and the average length of human summarization (based on footage) is 13.1% of the original video.
[0074] In all experiments, the automatic summarization results of our method were evaluated by comparing the algorithmic results (A) of our method with human-processed summaries (B) and obtaining scores (F), precision (P) and recall (R) ( A), as follows:
[0075]
[0076]
[0077]
[0078] Table 1 below shows the F-score scores of SumMe videos.
[0079] Table 1
[0080]
[0081]
[0082] Comparing the video summarization results obtained by this method with the...
PUM
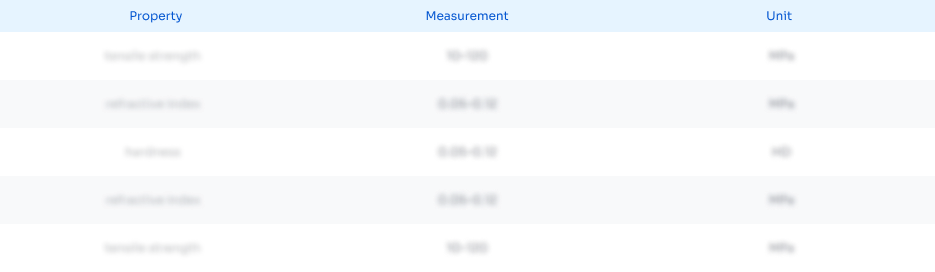
Abstract
Description
Claims
Application Information
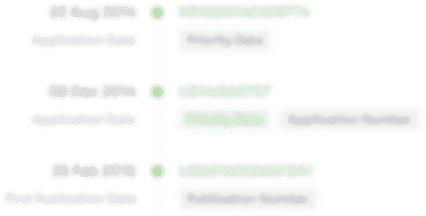
- R&D
- Intellectual Property
- Life Sciences
- Materials
- Tech Scout
- Unparalleled Data Quality
- Higher Quality Content
- 60% Fewer Hallucinations
Browse by: Latest US Patents, China's latest patents, Technical Efficacy Thesaurus, Application Domain, Technology Topic, Popular Technical Reports.
© 2025 PatSnap. All rights reserved.Legal|Privacy policy|Modern Slavery Act Transparency Statement|Sitemap|About US| Contact US: help@patsnap.com