Generative adversarial network-based pixel-level portrait cutout method
A pixel-level, network technology, applied in the field of computer vision, can solve the problems of time-consuming and labor-intensive data sets, large data set calibration and production costs, and small data sets, so as to enhance robustness, improve segmentation smoothness, The effect of high segmentation accuracy
- Summary
- Abstract
- Description
- Claims
- Application Information
AI Technical Summary
Problems solved by technology
Method used
Image
Examples
Embodiment 1
[0027] The present invention aims at the problem of inefficiency caused by the need for a large number of training set images in the existing portrait matting method, conducts research and innovation, and proposes a pixel-level portrait matting method based on a generative confrontation network. For real images containing portraits, like the fully convolutional network training model, the present invention also needs to obtain labeled images that separate real portraits and backgrounds by manual labeling, but the number of images in the training set is much smaller. see Figure 7 b, This is a human-annotated portrait and background separation image. see Figure 6 , the portrait matting method of the present invention comprises the following steps:
[0028] (1) Preset network: Preset the generation network and the discriminant network, and set the two networks to the confrontation learning mode, that is, the loss function of the generative network is obtained through the loss...
Embodiment 2
[0036]The overall technical scheme of the pixel-level portrait matting method based on generating confrontation network is the same as that in embodiment 1, the generating network described in step 1 is a deep neural network with skip connections, and the deep neural network with skip connections is used to form the generating network The gradient transfer path of the skip connection (Skip Connection) between the N serial encoder layers and the N serial decoder layers, which is also an identity mapping, see figure 1 , specifically connecting the 3-8 layer encoder layer to the 11-16 layer decoder layer, for example, the output of the 3rd layer encoder layer is simultaneously input to the 4th layer encoder layer and the 16th layer decoder layer, wherein, The output of the 3rd layer encoder layer to the 4th layer encoder layer is the basic output, and the output of the 3rd layer encoder layer to the 16th layer decoder layer is the result of the skip connection, and so on, forming...
Embodiment 3
[0038] The overall technical scheme of the pixel-level portrait matting method based on the generative confrontation network is the same as that of Embodiment 1-2. In the present invention, a random deactivation mechanism (Dropout) is introduced into the decoder layer of the generative network, specifically in the decoder of the generative network The inactivation parameters are randomly discarded before the final output of the layer, that is, each decoder layer of the generation network randomly throws away the respective inactivation parameters before the final output, specifically, the value of the respective inactivation part parameter output is randomly set to 0 , which simplifies many unnecessary calculations and ensures the robustness of the network structure.
PUM
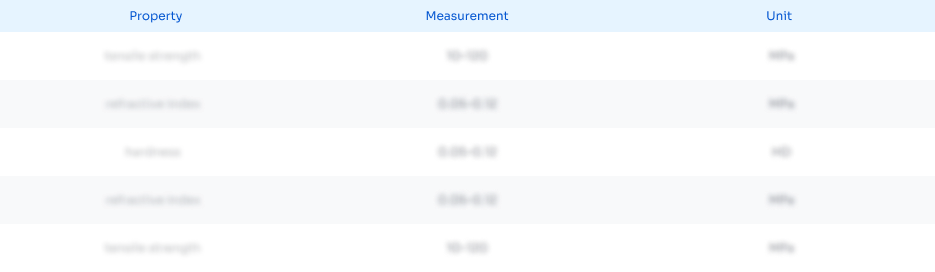
Abstract
Description
Claims
Application Information
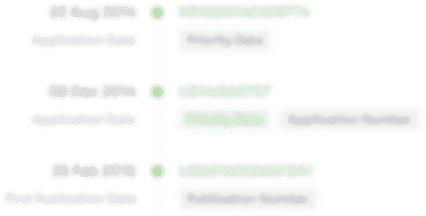
- Generate Ideas
- Intellectual Property
- Life Sciences
- Materials
- Tech Scout
- Unparalleled Data Quality
- Higher Quality Content
- 60% Fewer Hallucinations
Browse by: Latest US Patents, China's latest patents, Technical Efficacy Thesaurus, Application Domain, Technology Topic, Popular Technical Reports.
© 2025 PatSnap. All rights reserved.Legal|Privacy policy|Modern Slavery Act Transparency Statement|Sitemap|About US| Contact US: help@patsnap.com