Target-domain classifier training method, sample recognition method, terminal and storage medium
A training method and a recognition method technology, which are applied in the fields of terminals and storage media, target domain classifier training methods, and sample recognition methods, and can solve the problem of high cost of speech recognition.
- Summary
- Abstract
- Description
- Claims
- Application Information
AI Technical Summary
Problems solved by technology
Method used
Image
Examples
no. 1 example
[0056] In the prior art, when creating a classifier for the target domain, it is necessary to collect a large amount of sample data and mark these sample data before training the classification used to classify and identify unclassified samples in the target domain based on the marked samples. classifier, which is expensive to train. Especially in the field of speech recognition, due to the problem of different speech habits in different regions, it is difficult to create a separate speech classifier for each region. To address the above problems, this embodiment provides a target domain classifier training method. Combine below figure 1 The training method of the target domain classifier is described as follows:
[0057] S102. Perform feature extraction on the training samples to obtain training data.
[0058] In this embodiment, the training samples include two parts: one part is the samples from the source domain, which are called auxiliary samples here, and all samples i...
no. 2 example
[0080] The target domain classifier training method and sample recognition method provided in the foregoing embodiments are applicable to handwritten digital image samples or voice samples. This embodiment will continue to introduce the target domain classifier training method and sample identification method on the basis of the first embodiment, please refer to image 3 :
[0081] S302. Perform feature extraction on the training samples to obtain training data.
[0082] In this embodiment, the terminal uses the RBM algorithm to extract features from the training samples. Combine below Figure 4 A brief introduction to the principle of the RBM algorithm, Figure 4 A schematic diagram of the RBM model shown:
[0083] RBM generally consists of a two-layer structure of visible layer unit v and hidden layer unit h. The connection weight between the visible layer unit and the hidden layer unit is represented by w, the visible layer bias is represented by b, and the hidden layer...
no. 3 example
[0139] This embodiment firstly provides a storage medium, where one or more computer programs are stored. In an example of this embodiment, a target domain database construction program is stored in the storage medium, and the program can be read, compiled, and executed by a processor, so as to implement the flow of the target domain classifier training method in the foregoing embodiments. In other examples of this embodiment, a sample identification program may be stored in the storage medium, and the sample identification program may be executed by a processor to implement the sample identification method in the foregoing embodiments.
[0140] In addition, this embodiment also provides a terminal, please refer to Image 6 , the terminal 60 includes a processing 61 , a memory 62 and a communication bus 63 . Wherein the communication bus 63 is used to realize the connection and communication between the processor 61 and the memory 62, the memory 62 is used as a computer-reada...
PUM
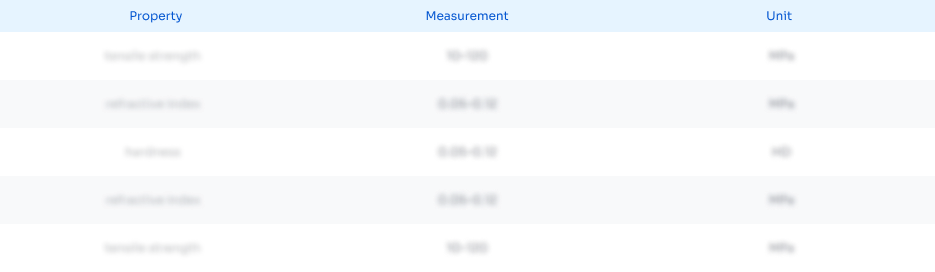
Abstract
Description
Claims
Application Information
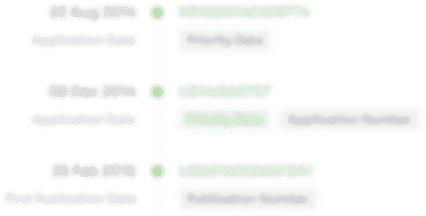
- R&D Engineer
- R&D Manager
- IP Professional
- Industry Leading Data Capabilities
- Powerful AI technology
- Patent DNA Extraction
Browse by: Latest US Patents, China's latest patents, Technical Efficacy Thesaurus, Application Domain, Technology Topic, Popular Technical Reports.
© 2024 PatSnap. All rights reserved.Legal|Privacy policy|Modern Slavery Act Transparency Statement|Sitemap|About US| Contact US: help@patsnap.com