Behavior identification method based on long-time deep time-space network
A space-time network and recognition method technology, applied in the field of image recognition, can solve problems such as difficulties in data collection and annotation, limited size and diversity, etc., and achieve the effect of improving recognition rate and robustness
- Summary
- Abstract
- Description
- Claims
- Application Information
AI Technical Summary
Problems solved by technology
Method used
Image
Examples
Embodiment 1
[0025] see figure 1 , a behavior recognition method based on a long-term deep spatio-temporal network, including the following steps:
[0026] S1. Build a multi-channel feature splicing network MFCN (Multi-Chunnel Feature Connected Network) model;
[0027] S2, select the video behavior data set, extract the video frame and optical flow frame of each video in the video behavior data set, and use the collection of video frames as the color image sequence data set I rgb , a collection of optical flow frames as an optical flow image sequence dataset I flowx , I flowy ;
[0028] S3, the color image sequence data set I rgb and Optical Flow Image Sequence Dataset I flowx , I flowy According to the continuous multi-frames, it is divided into several segments, and the segments are input into the multi-channel feature splicing network model. First, the spatio-temporal features of the continuous frames of each segment are extracted through the low-level convolutional layer, and the...
PUM
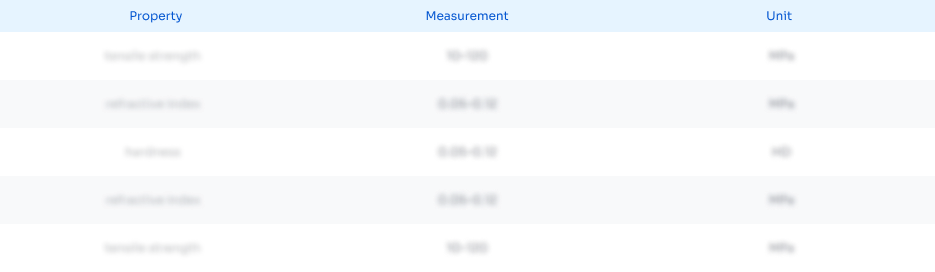
Abstract
Description
Claims
Application Information
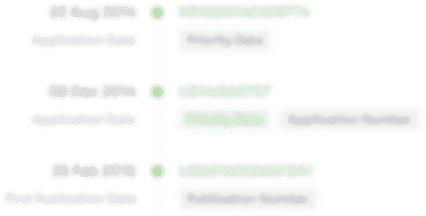
- R&D Engineer
- R&D Manager
- IP Professional
- Industry Leading Data Capabilities
- Powerful AI technology
- Patent DNA Extraction
Browse by: Latest US Patents, China's latest patents, Technical Efficacy Thesaurus, Application Domain, Technology Topic, Popular Technical Reports.
© 2024 PatSnap. All rights reserved.Legal|Privacy policy|Modern Slavery Act Transparency Statement|Sitemap|About US| Contact US: help@patsnap.com