Steel plate surface defect detection method based on multistage characteristics of convolutional neural network
A convolutional neural network and defect detection technology, which is applied in the field of steel surface defect detection based on multi-level features of convolutional neural network, can solve problems such as insufficient classification ability, lack of corresponding data, and inability to obtain accurate defect locations.
- Summary
- Abstract
- Description
- Claims
- Application Information
AI Technical Summary
Problems solved by technology
Method used
Image
Examples
Embodiment Construction
[0062] The specific implementation manners of the present invention will be further described in detail below in conjunction with the accompanying drawings and embodiments. The following examples are used to illustrate the present invention, but are not intended to limit the scope of the present invention.
[0063] Steel plate surface defect detection method based on multi-level features of convolutional neural network, such as figure 1 shown, including the following steps:
[0064] Step 1. Select an appropriate benchmark network, and then use the large dataset ImageNet to pre-train the benchmark network;
[0065] The benchmark network selects highly modular residual networks ResNet34 and ResNet50, both of which include the first convolutional layer conv1, four residual modules {R2, R3, R4, R5} and subsequent global maximum pooling layer and classification output layer; the difference between the two residual networks is that the number of convolutional layers and convolutio...
PUM
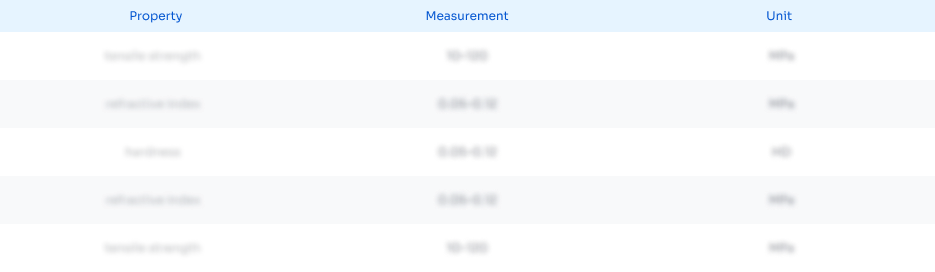
Abstract
Description
Claims
Application Information
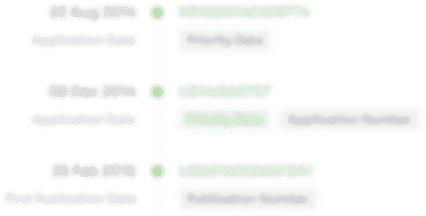
- R&D Engineer
- R&D Manager
- IP Professional
- Industry Leading Data Capabilities
- Powerful AI technology
- Patent DNA Extraction
Browse by: Latest US Patents, China's latest patents, Technical Efficacy Thesaurus, Application Domain, Technology Topic, Popular Technical Reports.
© 2024 PatSnap. All rights reserved.Legal|Privacy policy|Modern Slavery Act Transparency Statement|Sitemap|About US| Contact US: help@patsnap.com