Multi-mark learning method
A multi-label learning and multi-label technology, applied in the field of multi-label learning, achieves the effect of overcoming excessive feature dimension, strong generalization ability, and simple training
- Summary
- Abstract
- Description
- Claims
- Application Information
AI Technical Summary
Problems solved by technology
Method used
Image
Examples
Embodiment Construction
[0049] In order to make the purpose, technical solution, design method and advantages of the present invention clearer, the present invention will be further described in detail through specific embodiments in conjunction with the accompanying drawings. It should be understood that the specific embodiments described here are only used to explain the present invention, not to limit the present invention.
[0050] According to an embodiment of the present invention, a multi-label learning method is provided. In short, the method includes a process of training a multi-label learning model and a process of using the trained model to predict a label set of a new instance.
[0051] see figure 1 As shown, the training process of the multi-label learning model of an embodiment of the present invention includes the following steps:
[0052] Step S110, initialize a multi-label learning model including an autoencoder and a multi-classification model.
[0053] The multi-label learning m...
PUM
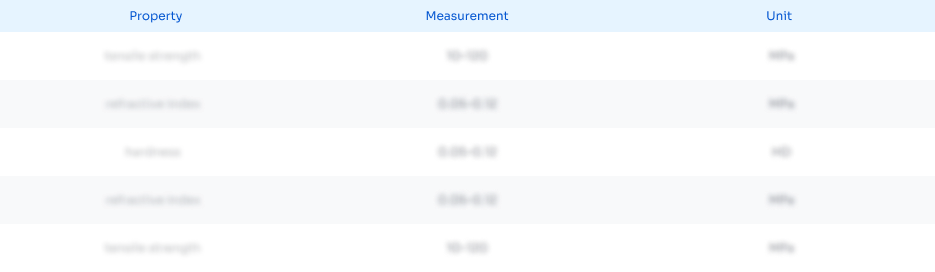
Abstract
Description
Claims
Application Information
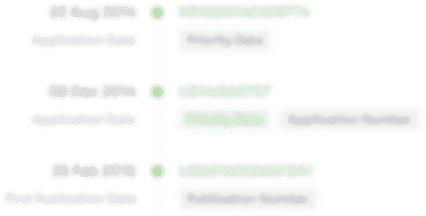
- R&D Engineer
- R&D Manager
- IP Professional
- Industry Leading Data Capabilities
- Powerful AI technology
- Patent DNA Extraction
Browse by: Latest US Patents, China's latest patents, Technical Efficacy Thesaurus, Application Domain, Technology Topic, Popular Technical Reports.
© 2024 PatSnap. All rights reserved.Legal|Privacy policy|Modern Slavery Act Transparency Statement|Sitemap|About US| Contact US: help@patsnap.com