Rapid path planning method based on variant dual DQNs (deep Q-networks) and mobile robot
A mobile robot and fast path technology, applied to road network navigators and other directions, can solve problems such as overestimation of action values and unsatisfactory mobile robots
- Summary
- Abstract
- Description
- Claims
- Application Information
AI Technical Summary
Problems solved by technology
Method used
Image
Examples
Embodiment Construction
[0043] Embodiments of the present invention are described in detail below, examples of which are shown in the drawings, wherein the same or similar reference numerals designate the same or similar elements or elements having the same or similar functions throughout. The embodiments described below by referring to the figures are exemplary only for explaining the present invention and should not be construed as limiting the present invention.
[0044] see figure 1 , the embodiment of the present invention is a mutation-based dual DQN fast path planning method, which includes the following steps:
[0045] Step S1: The mobile robot samples mini-batch transformation information (s, a, r, s′, d) from the experience playback memory, and randomly selects one of the two dueling deep convolutional neural networks according to the first preset rule as the first online network and the other as the first target network,
[0046] Wherein, the mini-batch is the number of sampling experien...
PUM
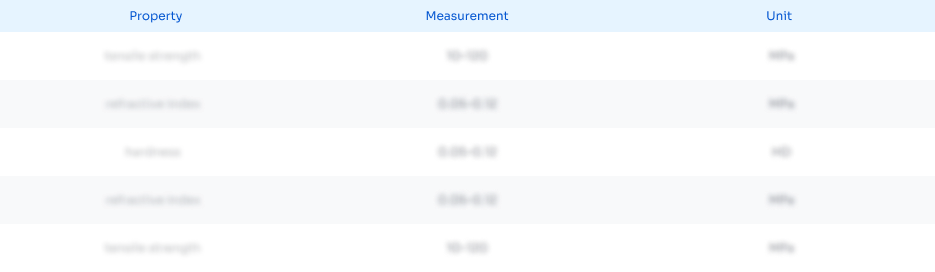
Abstract
Description
Claims
Application Information
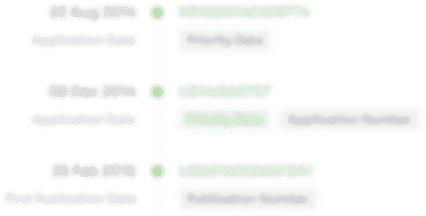
- R&D Engineer
- R&D Manager
- IP Professional
- Industry Leading Data Capabilities
- Powerful AI technology
- Patent DNA Extraction
Browse by: Latest US Patents, China's latest patents, Technical Efficacy Thesaurus, Application Domain, Technology Topic, Popular Technical Reports.
© 2024 PatSnap. All rights reserved.Legal|Privacy policy|Modern Slavery Act Transparency Statement|Sitemap|About US| Contact US: help@patsnap.com