Customer classification method and device based on cost sensitivity and semi-supervised classification
A cost-sensitive, classification method technology, applied in the field of customer classification methods and devices based on cost-sensitive and semi-supervised classification, can solve problems such as inability to mark categories, inability to determine whether to respond, overfitting, etc., to improve target customer selection performance , Good target customers choose the performance effect
- Summary
- Abstract
- Description
- Claims
- Application Information
AI Technical Summary
Problems solved by technology
Method used
Image
Examples
Embodiment 1
[0054] Such as figure 1 As shown, customer classification methods based on cost-sensitive and semi-supervised classification include:
[0055] S1. Obtain a dataset L with category labels, a dataset U without category labels, and a test set Test, and the number of initial samples in the dataset U without category labels is m.
[0056] S2. Using the random subspace method to train N basic classification models CS for the dataset L with category labels and the dataset U without category labels.
[0057] Described step S2 comprises:
[0058] S21. Selectively mark some samples from the unlabeled dataset U and add them to the labeled dataset L, and remove these samples from the unlabeled dataset U.
[0059] Described step S21 comprises:
[0060] S211. Set the threshold k, the threshold k represents the percentage of samples that you want to mark from the unclassified data set U in the unclassified data set U; calculate the samples of the selectively labeled sample set Q and the u...
Embodiment 2
[0071] Such as figure 2 As shown, the customer classification device based on cost-sensitive and semi-supervised classification includes data acquisition module, random subspace module, classification module and voting integration module.
[0072] The data acquisition module is used to obtain a data set L with class labels, a data set U without class labels and a test set Test, and the number of initial samples in the data set U without class labels is m.
[0073] The random subspace module is used to train N basic classification models CS by using the random subspace method on the dataset L with category labels and the dataset U without category labels.
[0074]The random subspace module includes a sample selective marker submodule and a random subspace submodule. The sample selective labeling submodule is used to selectively mark some samples from the unlabeled data set U and add them to the labeled data set L, and remove these samples from the unlabeled data set U. The r...
PUM
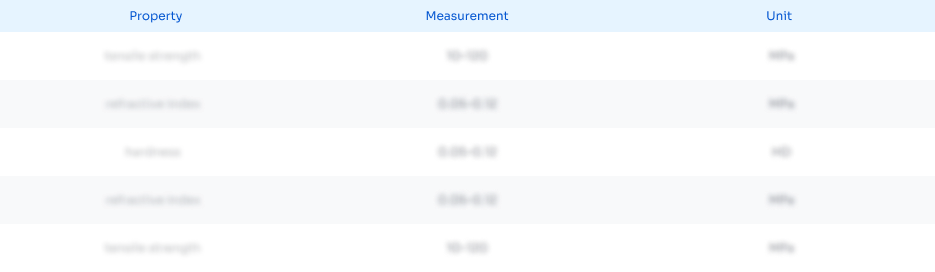
Abstract
Description
Claims
Application Information
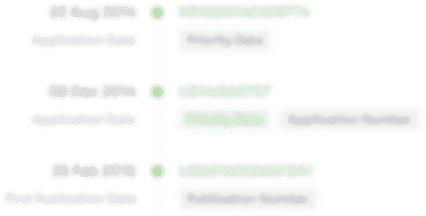
- R&D Engineer
- R&D Manager
- IP Professional
- Industry Leading Data Capabilities
- Powerful AI technology
- Patent DNA Extraction
Browse by: Latest US Patents, China's latest patents, Technical Efficacy Thesaurus, Application Domain, Technology Topic, Popular Technical Reports.
© 2024 PatSnap. All rights reserved.Legal|Privacy policy|Modern Slavery Act Transparency Statement|Sitemap|About US| Contact US: help@patsnap.com