Word vector and context information-based short text topic model
A topic model and word vector technology, applied in the field of short text topic models, can solve the problems of sparse word co-occurrence, low topic semantic consistency, and topic models cannot extract high-quality topics, so as to improve semantic consistency, Efficiency and effect improvement
- Summary
- Abstract
- Description
- Claims
- Application Information
AI Technical Summary
Problems solved by technology
Method used
Image
Examples
Embodiment Construction
[0021] The specific embodiments of the present invention will be described below to further illustrate the starting point and corresponding technical solutions of the present invention.
[0022] The present invention is a short text topic model based on word vectors and context information. The main purpose is to hope that the model can automatically extract high-quality topic information from short text data sets. The method is divided into the following four steps:
[0023] (1) Obtain the semantic similarity between words:
[0024] First, use Google’s open source tool word2vec to train the word vector from the Wikipedia dataset. If it is English training data, you need to use the English Wikipedia dataset to train the word vector. If it is Chinese training data, you need to use the Chinese Wikipedia dataset to train Chinese. The word vector of . Here, the English training data is taken as an example. The training data used are the Google review data set (Amazon Reviews) and...
PUM
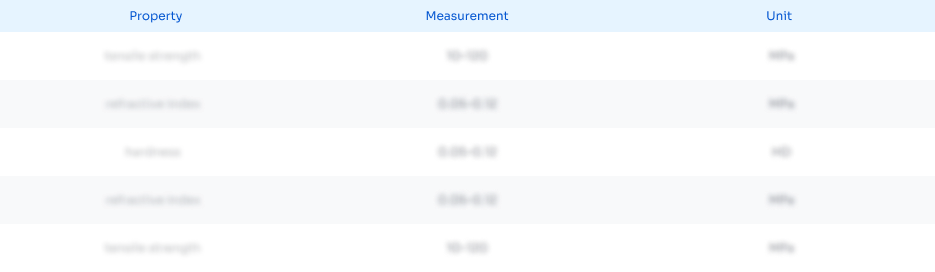
Abstract
Description
Claims
Application Information
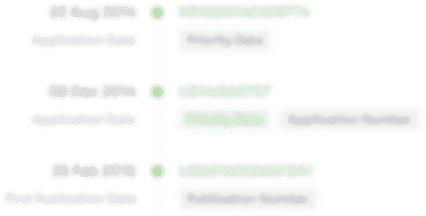
- R&D Engineer
- R&D Manager
- IP Professional
- Industry Leading Data Capabilities
- Powerful AI technology
- Patent DNA Extraction
Browse by: Latest US Patents, China's latest patents, Technical Efficacy Thesaurus, Application Domain, Technology Topic, Popular Technical Reports.
© 2024 PatSnap. All rights reserved.Legal|Privacy policy|Modern Slavery Act Transparency Statement|Sitemap|About US| Contact US: help@patsnap.com