Big data clustering algorithm for reducing risk of customer losing
A clustering algorithm and customer churn technology, applied in the field of clustering algorithms, can solve the problems of lack of big data clustering algorithm, inability to predict customer churn, etc., to achieve the effect of improving clustering accuracy and reducing the risk of inaccuracy
- Summary
- Abstract
- Description
- Claims
- Application Information
AI Technical Summary
Problems solved by technology
Method used
Image
Examples
Embodiment Construction
[0037] The specific implementation of the big data clustering algorithm for reducing the risk of customer loss disclosed by the present invention will be described in detail below in conjunction with the accompanying drawings, which is not intended to limit the scope of the present invention.
[0038] The present invention relates to the following theory:
[0039] (1) Axiomatic Fuzzy Sets (AFS). The AFS theory is a new semantic method for dealing with fuzzy information. Its essence is to study how to transform the internal laws or patterns contained in the training data or database into fuzzy sets and their logical operations. Member functions and their logical operations are determined by raw data and facts rather than intuition, imitating the mechanism by which human beings perceive and observe things to form concepts and generate logic, and discuss fuzzy concepts and their logical operations from a more abstract and general level. AFS theory mainly includes two parts: AFS ...
PUM
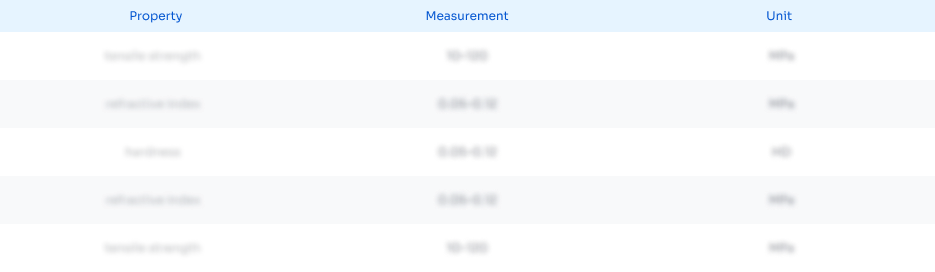
Abstract
Description
Claims
Application Information
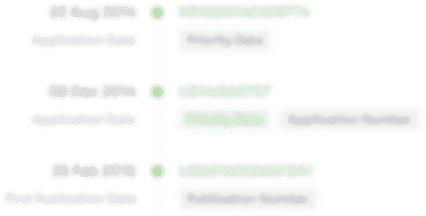
- R&D
- Intellectual Property
- Life Sciences
- Materials
- Tech Scout
- Unparalleled Data Quality
- Higher Quality Content
- 60% Fewer Hallucinations
Browse by: Latest US Patents, China's latest patents, Technical Efficacy Thesaurus, Application Domain, Technology Topic, Popular Technical Reports.
© 2025 PatSnap. All rights reserved.Legal|Privacy policy|Modern Slavery Act Transparency Statement|Sitemap|About US| Contact US: help@patsnap.com