Local similarity preserving-based hyperspectral image extreme learning machine clustering method
A hyperspectral image and extreme learning machine technology, applied in the field of hyperspectral image extreme learning machine clustering, can solve problems such as low clustering accuracy, no effective joint space-spectral information, and algorithm performance degradation.
- Summary
- Abstract
- Description
- Claims
- Application Information
AI Technical Summary
Problems solved by technology
Method used
Image
Examples
Embodiment
[0089] combine figure 1 , the implementation process of the present invention is described in detail below, and the steps are as follows:
[0090] Step 1: Reorganize the hyperspectral pixel matrix: input a hyperspectral image X 0 ∈ R D×W×H , taking the Selina_A data set with image band number D=204, image width W=86, and image height H=83 shown in Figure 4(a) as an experimental example.
[0091] The original hyperspectral data X 0 Arrange pixel by pixel to form matrix X=[x 1 ,x 2 ,...,x N ]∈R D×N As the input of the model, where N=W×H represents the number of hyperspectral pixels, x i ∈ R D Represents a hyperspectral pixel.
[0092] Step 2: Calculate the linear random response of hidden layer neurons: the specific process is as follows:
[0093] 2.1 Calculate the linear random response of the j-th hidden layer neuron to the i-th hyperspectral pixel:
[0094]
[0095] Among them, 1≤i≤N, w j =[w j1 ,w j2 ,...,w jD ]∈R D is a randomly generated weight vector, b...
PUM
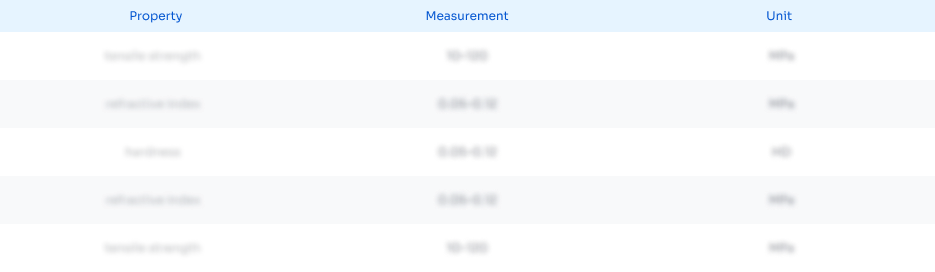
Abstract
Description
Claims
Application Information
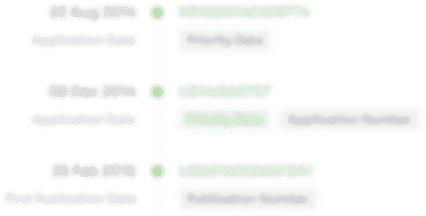
- R&D Engineer
- R&D Manager
- IP Professional
- Industry Leading Data Capabilities
- Powerful AI technology
- Patent DNA Extraction
Browse by: Latest US Patents, China's latest patents, Technical Efficacy Thesaurus, Application Domain, Technology Topic, Popular Technical Reports.
© 2024 PatSnap. All rights reserved.Legal|Privacy policy|Modern Slavery Act Transparency Statement|Sitemap|About US| Contact US: help@patsnap.com