Binary-channel convolutional neural network for facial expression recognition
A convolutional neural network, facial expression recognition technology, applied in biological neural network models, neural architecture, character and pattern recognition, etc. and other problems to achieve the effect of improving accuracy, improving accuracy, and enhancing expressive ability.
- Summary
- Abstract
- Description
- Claims
- Application Information
AI Technical Summary
Problems solved by technology
Method used
Image
Examples
Embodiment Construction
[0040] The present invention will be further described below in conjunction with the accompanying drawings, but the protection scope of the present invention is not limited thereto.
[0041] figure 1 The flow of facial expression recognition algorithm based on dual-channel convolutional neural network is given:
[0042]The human face facial expression recognition algorithm process that the present invention proposes comprises image preprocessing module and double-channel convolutional neural network (Binary Channel-Convolution Neural Network, BC-CNN), and the latter is extracted by double-channel feature network (Binary Channel-Convolution Neural Network, BC-CNN). Feature Extraction Network, BC-FEN) and weighted fusion classification network (Weighted Merge Classify Network, WMCN), can complete the feature extraction and expression classification of face images at the same time. The present invention preprocesses the input face image, including face detection, rotation correc...
PUM
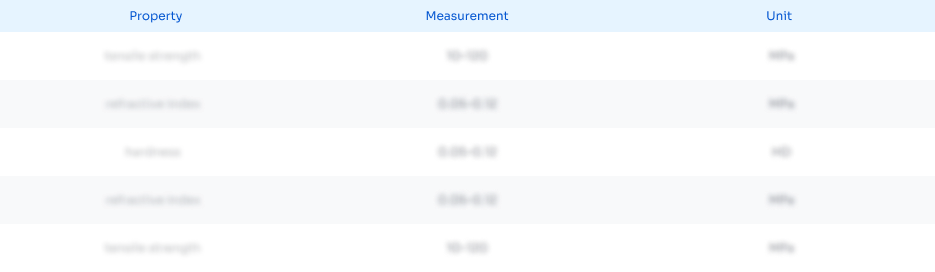
Abstract
Description
Claims
Application Information
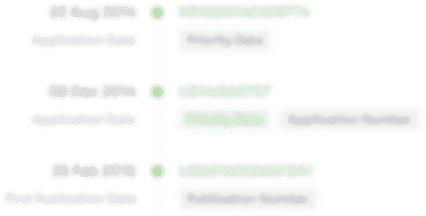
- R&D Engineer
- R&D Manager
- IP Professional
- Industry Leading Data Capabilities
- Powerful AI technology
- Patent DNA Extraction
Browse by: Latest US Patents, China's latest patents, Technical Efficacy Thesaurus, Application Domain, Technology Topic, Popular Technical Reports.
© 2024 PatSnap. All rights reserved.Legal|Privacy policy|Modern Slavery Act Transparency Statement|Sitemap|About US| Contact US: help@patsnap.com