Classification method based on Triple-GAN
A classification method and classifier technology, applied in the field of image processing, can solve problems such as gradient disappearance, uneven labeling, unstable model training, etc., and achieve the effect of strong adaptability
- Summary
- Abstract
- Description
- Claims
- Application Information
AI Technical Summary
Problems solved by technology
Method used
Image
Examples
Embodiment
[0101] Now widely used dataset MNIST. Among them, MNIST includes 60,000 training samples, 10,000 validation samples and 10,000 test samples of handwritten digits with a size of 28×28.
[0102] (1) The MNIST dataset is available on Kaggle. After downloading the train.csv to the data / folder, load the file into the training data.
[0103] (2) The generator generates sample data marked with pseudo-labels according to the training data.
[0104] Input the 10,000 verification sample data set of MNIST into the classifier (C), establish a decision tree with a depth of M according to the random forest algorithm based on the binary decision tree, set the height of the decision tree to 5, and establish 304 binary decisions Trees, respectively built binary decision tree label labels.
[0105] (3) The classifier predicts the joint distribution of samples and class labels:
[0106] 60,000 MNIST training data sets are input into the generator, and the generated data x and class label y ...
PUM
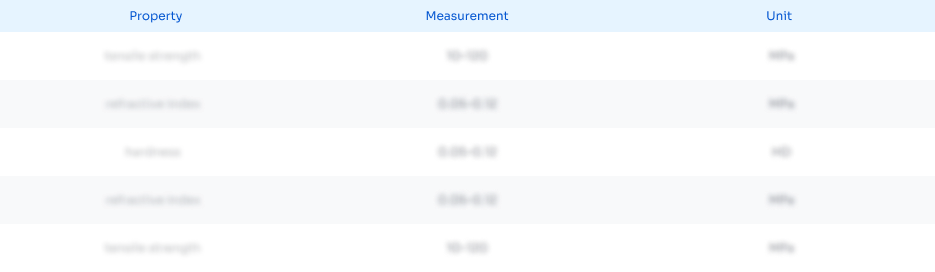
Abstract
Description
Claims
Application Information
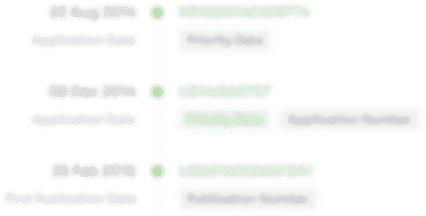
- R&D
- Intellectual Property
- Life Sciences
- Materials
- Tech Scout
- Unparalleled Data Quality
- Higher Quality Content
- 60% Fewer Hallucinations
Browse by: Latest US Patents, China's latest patents, Technical Efficacy Thesaurus, Application Domain, Technology Topic, Popular Technical Reports.
© 2025 PatSnap. All rights reserved.Legal|Privacy policy|Modern Slavery Act Transparency Statement|Sitemap|About US| Contact US: help@patsnap.com