Sample class classification method of atom Laplacian regularization-based semi-supervised dictionary learning
A dictionary learning, semi-supervised technology, applied in character and pattern recognition, instruments, computer parts, etc., can solve problems such as less labeled data and the effect of dictionary classification, to ensure simplicity, fast and effective dictionary learning, implementation The effect of constant alternating updates and optimizations
- Summary
- Abstract
- Description
- Claims
- Application Information
AI Technical Summary
Problems solved by technology
Method used
Image
Examples
Embodiment Construction
[0033] In order to make the object, technical solution and advantages of the present invention clearer, the present invention will be further described in detail below in conjunction with the accompanying drawings.
[0034] Such as Figure 1 to Figure 2 As shown, in the embodiment of the present invention, the present invention is a semi-supervised dictionary learning method based on atomic Laplacian graph regularization. The hardware and programming language for the specific operation of the method of the present invention are not limited, and can be written in any language All can be completed, so other working modes will not be described in detail.
[0035] Embodiments of the present invention adopt a computer with Intel Xeon-E5 central processing unit and 16G byte internal memory, and have compiled the work program based on the semi-supervised dictionary learning of atomic Laplacian graph regularization with Matlab language, have realized this method of invention.
[003...
PUM
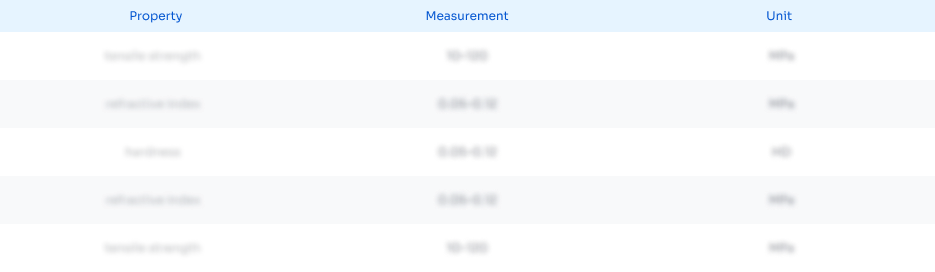
Abstract
Description
Claims
Application Information
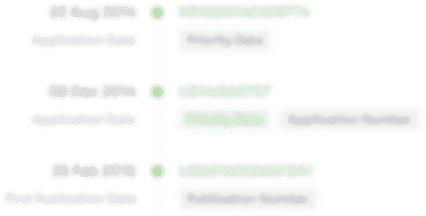
- R&D Engineer
- R&D Manager
- IP Professional
- Industry Leading Data Capabilities
- Powerful AI technology
- Patent DNA Extraction
Browse by: Latest US Patents, China's latest patents, Technical Efficacy Thesaurus, Application Domain, Technology Topic, Popular Technical Reports.
© 2024 PatSnap. All rights reserved.Legal|Privacy policy|Modern Slavery Act Transparency Statement|Sitemap|About US| Contact US: help@patsnap.com