A personalized diet recommendation method for diabetes mellitus by introducing adaboost probability matrix decomposition
A technology of probability matrix decomposition and recommendation method, which is applied in the field of diabetes personalized diet recommendation by introducing Adaboost probability matrix decomposition, which can solve the problems of food infiltration, inability to eat, and ignoring the particularity of patients.
- Summary
- Abstract
- Description
- Claims
- Application Information
AI Technical Summary
Problems solved by technology
Method used
Image
Examples
Embodiment
[0041] The recommended method of the present invention specifically comprises the following steps:
[0042] Step 1. Set the dietary preference feature set of diabetic patients as U={u 1 ,u 2 ,...,u n} and the attribute feature set of food is V={v 1 ,v 2 ,...,v m},As shown in Table 1;
[0043] Table 1 Correlation degree between diabetic patients and food characteristics
[0044]
[0045]
[0046] Among them, there are n rows in the matrix representing the n dietary preference characteristics of a diabetic patient, and m columns representing the attribute characteristics of the food; assuming that a diabetic patient has a behavior on a food, the value of r will be distributed in the matrix , through the calculation of r to determine whether the relationship between diabetic patients and food satisfies both individuality and medical indicators.
[0047] Take the characteristic attributes of an overweight diabetic patient: weight (overweight), taste preference (love t...
PUM
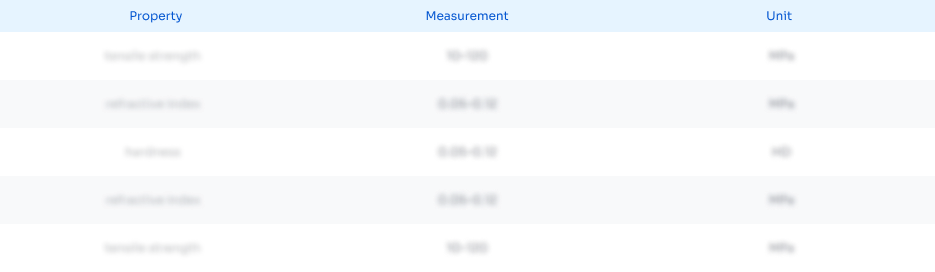
Abstract
Description
Claims
Application Information
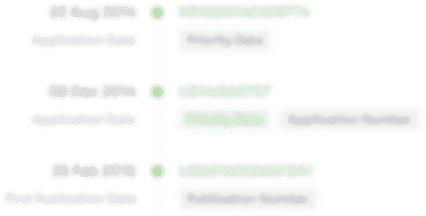
- R&D
- Intellectual Property
- Life Sciences
- Materials
- Tech Scout
- Unparalleled Data Quality
- Higher Quality Content
- 60% Fewer Hallucinations
Browse by: Latest US Patents, China's latest patents, Technical Efficacy Thesaurus, Application Domain, Technology Topic, Popular Technical Reports.
© 2025 PatSnap. All rights reserved.Legal|Privacy policy|Modern Slavery Act Transparency Statement|Sitemap|About US| Contact US: help@patsnap.com