Image hash code training model algorithm and classification learning method based on binary weight
A technology for training models and learning methods, which is applied in computing, computer components, character and pattern recognition, etc., and can solve problems such as poor classification effect, high computing overhead, and high memory usage
- Summary
- Abstract
- Description
- Claims
- Application Information
AI Technical Summary
Problems solved by technology
Method used
Image
Examples
Embodiment 1
[0067] An image hash code training model algorithm based on binary weights, the algorithm includes the following steps:
[0068] Step 1.1. Select the exponential loss function as the loss function used, and the formula of the objective equation is:
[0069]
[0070]
[0071] Let the generated image binary code be b i is the original training dataset middle x i For the corresponding r-bit binary code, a linear hash equation is set as:
[0072] b=sgn(P T x)
[0073] here P is the image hash transpose matrix; T is the transpose symbol; d is the dimension of image x; r is the hash code length;
[0074] The binary code of the classifier is w.
[0075] Step 1.2. Perform unified learning on the classifier obtained in step 1.1 and the binary code of the training image features, update the hash code of the training image features and the binary code of the classifier, optimize the objective equation of the loss function selected in step 1.1, and get optimized After th...
Embodiment 2
[0106] On the basis of Embodiment 1, different loss functions are selected, and the steps of a hash code image training model algorithm based on binary weights are as follows:
[0107] Step 1.1. Select a simple linear loss function as the loss function used, and the formula of the objective equation is:
[0108]
[0109]
[0110] Let the generated image binary code be b i is the original training dataset middle x i For the corresponding r-bit binary code, a linear hash equation is set as:
[0111] b=sgn(P T x)
[0112] here P is the image hash transpose matrix; T is the transpose symbol; d is the dimension of image x; r is the hash code length;
[0113] The binary code of the classifier is w.
[0114] Step 1.2: Perform unified learning on the classifier obtained in step 1.1 and the binary code of the training image feature, update the hash code of the training image feature and the binary code of the classifier, optimize the objective equation of the loss func...
PUM
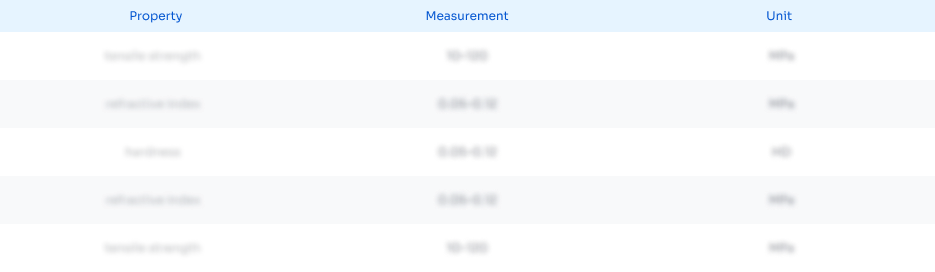
Abstract
Description
Claims
Application Information
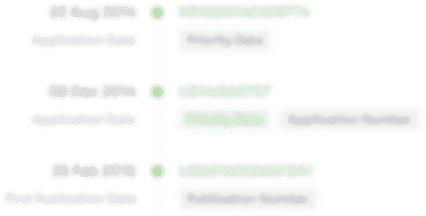
- R&D Engineer
- R&D Manager
- IP Professional
- Industry Leading Data Capabilities
- Powerful AI technology
- Patent DNA Extraction
Browse by: Latest US Patents, China's latest patents, Technical Efficacy Thesaurus, Application Domain, Technology Topic, Popular Technical Reports.
© 2024 PatSnap. All rights reserved.Legal|Privacy policy|Modern Slavery Act Transparency Statement|Sitemap|About US| Contact US: help@patsnap.com