Multi-target tracking method based on LSTM network and deep reinforcement learning
A multi-target tracking and reinforcement learning technology, applied in the field of multi-target tracking based on LSTM network and deep reinforcement learning, can solve the problems of insufficient model and inaccurate tracking results, so as to overcome the lack of comprehensive model, improve the accuracy and many more. Effect of Target Tracking Accuracy
- Summary
- Abstract
- Description
- Claims
- Application Information
AI Technical Summary
Problems solved by technology
Method used
Image
Examples
Embodiment Construction
[0025] The present invention will be further described below in conjunction with the accompanying drawings and specific embodiments.
[0026] Such as figure 1 As shown, the multi-target tracking method based on LSTM network and deep reinforcement learning includes the following steps:
[0027] (1) Use YOLO V2 target detector to detect each frame of image in the video to be tested, output the detection result, set the detection result of the tth frame image as a set is the jth detection result of the tth frame image, and N is the total number of detections;
[0028] (2) if figure 2 As shown, multiple single-target trackers based on deep reinforcement learning technology are constructed, each single-target tracker includes a convolutional neural network CNN and a fully connected layer FC, and the convolutional neural network is built on the basis of the VGG-16 network , VGG-16 belongs to the state-of-the-art and has wide applications in deep learning methods. The CNN net...
PUM
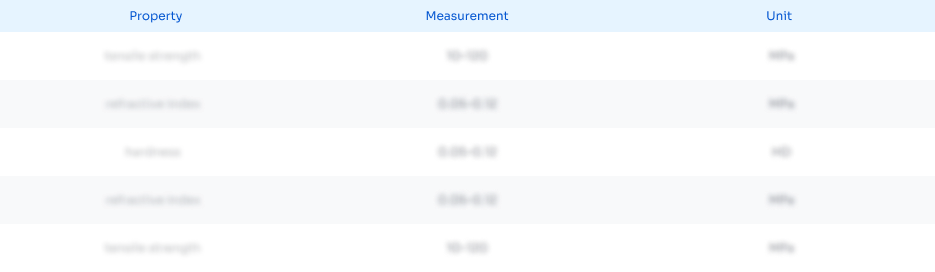
Abstract
Description
Claims
Application Information
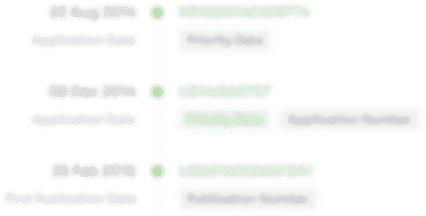
- R&D Engineer
- R&D Manager
- IP Professional
- Industry Leading Data Capabilities
- Powerful AI technology
- Patent DNA Extraction
Browse by: Latest US Patents, China's latest patents, Technical Efficacy Thesaurus, Application Domain, Technology Topic.
© 2024 PatSnap. All rights reserved.Legal|Privacy policy|Modern Slavery Act Transparency Statement|Sitemap